Lieutenant Colonel Alessandro Nalin, Italian Army; and Paolo Tripodi, PhD
https://doi.org/10.21140/mcuj.20231401003
PRINTER FRIENDLY PDF
EPUB
AUDIOBOOK
Abstract: The application of artificial intelligence (AI) technology for military use is growing fast. As a result, autonomous weapon systems have been able to erode humans’ decision-making power. Once such weapons have been deployed, humans will not be able to change or abort their targets. Although autonomous weapons have a significant decision-making power, currently they are not able to make ethical choices. This article focuses on the ethical implications of AI integration in the military decision-making process and how the characteristics of AI systems with machine learning (ML) capabilities might interact with human decision-making protocols. The authors suggest that in the future, such machines might be able to make ethical decisions that resemble those made by humans. A detailed and precise classification of AI systems, based on strict technical, ethical, and cultural parameters would be critical to identify which weapon is suitable and the most ethical for a given mission.
Keywords: artificial intelligence, AI, machine learning, ML, lethal autonomous weapon systems, decision making, military ethics, commander responsibility
The application of AI technology for military use is growing fast. AI technology already supports several new systems and platforms, both kinetic and nonkinetic (e.g., autonomous drones with explosive payloads or cyberattacks). Although the human role remains extremely important in the deployment of such weapons, the increasing use of AI has made weapons able to erode humans’ decision-making power. Humans have full control of semiautonomous weapon systems. They have partial control, but ultimately retain the ability to override supervised autonomous systems and finally have no control on unsupervised autonomous systems. In the last case, once these systems have been deployed, humans will not be able to change or abort their targets.
Probably the most controversial weapons are the unsupervised autonomous weapons as humans have no ability to control them once they have been deployed. Although the number of such weapons available today is limited, very likely autonomous weapons will continue to be developed and their applicability will expand.1
The debate among scholars and practitioners about the use of these weapon systems focuses mainly on their potential targets; however, in this article the authors suggest that we should look at the use of these weapon systems as part of a mission. Semi- and supervised autonomous weapon systems will be deployed by military operators in support of a mission while autonomous weapons will be given a mission to accomplish. The central issue we deal with is that, although autonomous weapons might be empowered with accomplishing a mission and therefore will have a significant decision-making power, very likely they will not be able to make ethical choices. Indeed, what is still missing in the autonomous weapons systems is the ability to explore and consider alternative courses of action if the assigned mission might have unforeseen, unethical consequences. For example, after a loitering munition has been given a mission to destroy a radar station, at the critical moment of attack, a civilian vehicle with a family might be passing by the target. The autonomous weapon system will continue on its mission and might ignore any possible unethical collateral damage.
As a result, the deployment of AI in the battlefield has generated an important debate about the responsibility gap problem. In relation to the use of lethal autonomous weapon systems (LAWS), Ann-Katrien Oimann provides a detailed exploration of the current debate about the responsibility gap problem. She identifies two main positions supported by those who believe that the responsibly gap problem can be filled through the adoption of three different approaches (technical solution, practical arrangement, and holding the system responsible), and those who believe that the problem is unsolvable.2 In the authors’ view there is an approach that, to a certain extent, has the potential to bridge these apparently irreconcilable positions. In fact, a detailed and precise classification of AI systems, based on strict parameters, would be critical to identify which weapon is suitable for a given mission. These parameters might be of a technical, ethical, and cultural nature. For example, suppose that a specific AI system that possesses the right features to perform a given mission is available. In that case, the chain of command that deliberately uses a different AI system should be held responsible for the potentially unethical outcome. However, if the correct AI system is used, but it makes a decision that results in an unexpected and unethical outcome, the event should be considered as if the decision had been made by a human in good faith. This last case makes evident why the cultural factor, intended as the human willingness to accept AI’s potentially harmful decision, will play a decisive role in AI integration in the human decision-making process.
The fact that today’s autonomous weapons cannot make ethical choices does not necessarily mean that they will never be able to decide ethically. The authors suggest that in the future such machines might be able to make ethical decisions that resemble those made by humans.
Therefore, it is essential to explore and determine as much as possible which machine decisions, and in particular ethical decisions, could be acceptable for humans. Failing to do so might have two implications. On the one hand, those who are overconfident in AI systems might be inclined to accept all automated decisions, believing that computers are much sharper than human beings. On the other hand, those who are skeptical about computer decisions, as they believe that computers do not ever meet sufficient ethical standards, might not accept any AI-generated decision. It is possible that they would give up the potential benefits that this technology might offer to military decision making in terms of speed and accuracy. The challenge is to develop an approach to AI-driven decision making that identifies a middle ground between the overconfident and skeptical camps.
The parameters used to identify such an intermediate point will consider first the available technology, second the ethical framework, and finally the human predisposition in accepting AI’s decisions. This article focuses on the possible ethical implications of AI integration in the military decision-making process. It will explore how the particular characteristics of AI systems with machine learning (ML) capabilities might interact with human decision-making protocols.
The Technological Factor and the AI Galaxy
Autonomous technologies will continue to increase the role of AI, but even more so, they will rely on ML to be able to develop the ability to mimic human thinking and behavior. Currently, as much as AI tries to simulate human intelligence, it still lacks the human curiosity or initiative to learn how to do what it is not programmed for.3 Inside the AI field, it is important to note the role played by artificial narrow intelligence (ANI) systems that can perform tasks limited to a specific area (Google Maps can plot a route but cannot forecast weather); and artificial general intelligence (AGI) systems that resemble more closely human intelligence as it has “the ability to see the whole” in making decisions.4 While there are many examples of highly efficient ANI systems already available, currently it has proved to be impossible to develop a reliable AGI system to be used in support of decision-making processes.5 Therefore, this article will refer mainly to ANI, identifying them as AI systems. Regarding machine learning, James E. Baker defines it as “the capacity of a computer using algorithms, calculation, and data to learn to better perform programmed tasks and thus optimize functions.”6
Although capable of giving better, faster outputs while optimizing resources, machines that are programmed to play chess will never take the initiative to learn how to play a different game, for example checkers, because they are limited to perform those actions they are programmed for. The authors do not exclude the fact that AI systems might behave in unpredicted ways. This article is based on the concern that it might happen. That means if an AI system is programmed to perform a certain action (play a specific game) that system will improve its skill in playing that game, but it will not take the initiative to learn another game.
These machines are fundamentally reactive, yet they are becoming more proactive within the limits of their specific use. In the last two decades, integration of ML in some applications improved AI’s proactive attitude. Brian David Johnson rightly noted that we should expect that “all technologies will use AI and ML. The use of the term could become meaningless because AI and ML will be subsumed by software in general.”7 Consider, for example, Google Nest Thermostat; this home improvement gadget observes users’ behavior and pattern of life (e.g., what corrections has the user made in previous days? What time does the user leave home or come back?) to set temperature values in different moments of the day or week. Yet, this improvement in proactive attitude is still far from mirroring the curiosity of the human brain to explore and learn something new without having been directed to do so.
The integration of AI and ML will allow for the creation of machines that can mimic human brain behavior. Stuart J. Russel and Peter Norvig propose a taxonomy that categorizes AI based on the ability of these systems to “think rationally; act rationally; think like humans; and act like humans.”8 Machines will be able to think and act rationally, adopting criteria of a clear definition of what is rationally right and wrong.9 What is right and wrong follows a static course of action, therefore it is not going to change. The general expectation from these machines is that, given a specific set of inputs, outputs will remain the same over time. The limit of these systems emerges when they have to make decisions in situations in which there is no right or wrong model for answers. Machines that think and act like humans do not refer to rationality but try to behave like humans. This difference implies the possibility to learn from experience among all the other things. ML enables machines to learn from experience.10 However, if the reference model is based on true or false answers, they are not sufficient to replicate human behavior.
The complexity of human decision making requires an approach that should go beyond the binary logic of yes or no typical of a computer algorithm. Bahman Zohuri and Moghaddam Masoud analyze in details the concept of fuzzy logic: “an approach to computing based on ‘degrees of truth’ rather than the usual ‘true or false’ (1 or 0) Boolean logic on which the modern computer is based.”11 Fuzzy logic is fundamental for the building of effective AI systems as it processes decisions, categorizing them not only as entirely right or wrong but also on a continuum between these categories. Arguably, the combination of ML and fuzzy logic allows the creation of autonomous systems that can effectively mimic human reasoning and decision making in its peculiar ability to learn from experience and express judgment like, for example, almost right or not completely wrong.
The Ethical Factors: Ethical Aspects of the Integration of AI in Decision Making
AI systems act in the ethics realm, yet their qualification as ethical agents requires some consideration. James H. Moor provides an analysis of the nature of different machine ethics through the different typologies of ethical agency. He differentiates among machines that have implicit agency, machines whose inherent design prevents unethical behavior (i.e., “pharmacy software that checks for and reports on drug interactions”); explicit agency, machines able to “represent ethics explicitly and then operate effectively on the basis of this knowledge”; and full ethical agency, machines that possess “consciousness, intentionality, and free will.”12 At the moment, there are no machines that possess these three characteristics; however, according to Moor, AI systems are ethical enough to act as ethical agents, with all the necessary limitations for their specific functions.13 Humans can assess a machine’s ethics and employ it in its specific and limited sector when built for a particular purpose, like a tracking and triage system designed for disaster relief operations.14 Humans could trust completely the ethics of AI systems employed for unconstrained general purposes if they would achieve the status of full moral agency. Yet, “narrow” AI (ANI) is the only system currently available.
The use of AI systems to support self-driving vehicles has generated a valuable debate about how to integrate ethics in AI systems to develop their ability to make ethical decisions. Vincent Conitzer et al. found that, in this field, a rationalist ethical approach alone would probably lead to decisions that maximize utility but might not be entirely ethical.15 They suggested that initial rationalistic approaches should integrate later on a machine learning approach based on “human-labeled instances.”16 As a result, after a system has learned how to decide following a strictly rationalistic approach, humans should continue to feed such systems with information about what constitute a right ethical decision in a variety of different situations.
Noah Goodall, in “Ethical Decision Making During Automated Vehicles Crashes,” takes a similar approach, but with a more defined practical sequence of actions to better integrate ethics in AI systems for self-driving vehicles. Goodall identifies three phases in the development of ethical AI systems. In the first phase, vehicles use a rationalistic moral system (e.g., consequentialism) taking action to minimize the impact of a crash based on general outcomes (e.g., injuries are preferable to fatalities).17 In the second phase, while building on the rules established in the first phase, vehicles will learn how to make ethical decisions observing human choices across a range of real-world and simulated crash scenarios.18 The third and final phase requires an automated vehicle to explain its decisions using “natural language” so that humans may understand and correct its highly complex and potentially incomprehensible-to-humans logic.19 This ability will help humans understand why vehicles make certain and maybe unexpected choices, and developers will be able to understand and, more importantly, correct wrong behaviors and decisions.20
Conitzer et al. and Goodall concur on a phased AI training that begins with the implementation of a consequentialist approach and continues with the integration of human-based experience and expertise.21 The fast development of technological improvements leads us to believe that probably soon we will be able to build an effective ethical framework in AI systems. Developers can build ethics into AI systems adopting either a top-down or a bottom-up approach. With the former, developers will code into AI systems all the desired ethical principles (i.e., “Asimov’s Three Laws of Robotics, the Ten Commandments or . . . Kant’s categorical imperative”).22 With the bottom-up approach, machines will learn from human behavior in multiple situations without a specific base of moral or ethical knowledge.23
With the top-down approach, it is not necessary to program all the possible decisions that machines might take in different situations as they will decide in line with their embedded principles.24 This approach highlights the importance of fuzzy logic implementation as it allows AI systems to go beyond the simple dichotomy of right and wrong.25 The possibility to make decisions that are sufficiently right or not completely wrong widens the range of possible choices in which humans can identify those acceptable to them. However, the moral strength of humans’ decisions is based on a lifelong ethical development that typically begins from childhood, while top-down AI’s ethics are passive to external changes. According to Amitai Etzioni and Oren Etzioni, a top-down approach is “highly implausible.”26
With the bottom-up approach, machines learn from human behavior in multiple situations without a specific base of moral or ethical knowledge.27 Machines observe how humans behave and react to situations. From these observations, machines create their set of rules to make decisions independently. The main concern with this approach is that humans are not flawless and make mistakes that AI systems may not recognize and consequently absorb as a model of behavior.28 It is apparent that both top-down and bottom-up approaches present flaws that can hamper the ethical competence of machines and are hard to mitigate.
Assuming that technology can support the development of a full moral agent AI, such a machine would be the most evolved AI system. The application of a top-down approach would mitigate the ability to learn mainly from experiences, because machines rely mostly on human-labeled data or instructions inputted by a limited number of individuals instead of having access to the entire human experience on a specific action/behavior. The bottom-up approach would expose the machine to the human’s natural flaws and misbehavior and will allow for the development of a machine that, like humans, might make mistakes. Arguably, this last scenario might lead humans to over rely on a system that, although similarly inaccurate as humans, could be more effective because of its speed and user friendliness.
An additional issue to consider is about perception of responsibility in relation to decisions made by AI systems. Due to their high level of autonomy to identify and engage military targets while pursuing their mission, the employment of autonomous weapon systems raises concerns about responsibility and accountability in cases of wrong decisions and actions.29 Mark Ryan raises the issue of responsibility of AI decisions, pointing out that if, on the one hand, it is unfair to assign the responsibility of wrong AI systems’ decisions to their designers because these systems can learn; on the other hand, AI cannot be responsible for its decisions because it is not a moral agent.30
Ross W. Bellaby takes into consideration those aspects related to the responsibility of military AI systems’ failures analyzing different cases involving autonomous weapons or remote-controlled weapons systems. He argues that responsibility goes together with the possibility of making decisions.31 The rationale is that if an ethical failure happens using a remote-controlled weapon, the human pilot or the human chain of command will be responsible for that failure. However, if an autonomous weapon system makes an ethical error, it would be its AI’s responsibility, but AI is not an entity subject to legal action, so the responsibility should go to its developers or to those who decide to employ that system for that mission. While developers might argue that they have written the code a long time before and without the information available at the moment of the failure, the human chain of command might also maintain that they cannot influence decisions and issue orders to avoid the failure.32 The identification, made on the most objective of bases, of which is the best AI for each specific situation would be a helpful tool to establish, at least if there is some responsibility for having resorted to the wrong AI system.
Eventually, the complete reliance on AI systems can create a gap in the responsibility and accountability chain that ultimately can “create distance from and mitigate the responsibility of the military operators or commanders using the system.”33 The risk is that humans, feeling themselves free from any responsibility, might fail to consider the ethical implication of decisions made by AI systems. However, in all those cases in which there is not a clear, unpredictable technological failure, the responsibility for mistakes made by a full autonomous weapon system while pursing the assigned mission should rest with its chain of command.
Future Battlefield Environments: Capitalizing Advantages of AI in the Decision-making Process
In the near future, combatants will confront enemies capable of conducting multidomain operations (MDOs) that will take place simultaneously in the air, land, maritime, space, and cyberspace. In MDOs, humans might find it difficult to make fast and, more importantly, timely decisions. It is in such environments that automated systems will be extremely beneficial to support the AI’s human-out-of-the-loop decision-making process.34 This support will be crucial to save time and gain an advantage on the enemy. Anupam Tiwari and Adarsh Tiwari noted that “often the timelines are dominated by the time it takes to move equipment or people or even just the time that munitions are moving to targets. It is important not to overstate the value of accelerating the decision process in these cases.”35 However, this approach does not consider the enduring nature of decision-making processes; once the headquarters issues its order and troops move on the battlefield, the observe-orient-decide-act (OODA loop) cycle keeps on running to maintain the order consistent with changes in the common operational picture. For this reason, AI systems might be far more relevant than it may appear.
Moreover, in relation to the OODA loop, there are similarities in the way machines and humans make decisions. For example, Amitai and Oren Etzioni state that autonomous vehicles “are programmed to collect information, process it, draw conclusions, and change the ways they conduct themselves accordingly, without human intervention or guidance.”36 These vehicles are programmed to approach the decision-making process in the same way militaries do through the OODA loop.
AI systems improve data collection and accelerate the elaboration and update of situational awareness. Operations are information-driven, and success often is on the side of those who possess better situational awareness of the battlefield. More information allows planners to predict enemies’ moves and, possibly, preempt them.37 It is reasonable to think that today’s significant AI limitations, for example its highly restricted “ability to recognize images (observe) outside of certain conditions” are transitory.38 In the future, AI will be able to improve intelligence collection consistently, increasing the sharpness of situational awareness by different applications like, for example, improved image, facial, voice recognition, aggregation of data, and translation.39 An indication of this future scenario is the U.S. Army development of the capability to deploy swarms of drones to “increase situational awareness with persistent reconnaissance.”40 More refined and vast amounts of information available in less time will allow those who possess this technology to have a decisive advantage over the opponent in terms of situational awareness at the beginning of the OODA loop cycle.
Automated instruments of data processing with the support of AI can provide better intelligence and suggest options for military problems. Genetics, culture, and consolidated expertise heavily influence each decision maker’s mental model to process information and produce intelligence.41 In simple words, the observation phase in a multidomain environment can quickly run out of humans’ analysis capabilities, reducing the speed at which military planners can make decisions and act.42 To mitigate the shortage of analysis capabilities, AI and ML systems help implement an out-of-the-loop system in which a human’s contribution is limited strictly to the necessary, which might be the activation of the system or definition of parameters used to identify an actor on the battlefield as foe.43 According to Daniel J. Owen, AI will play a prominent role in the transformation of “human decision-makers’ abilities to orient by integrating and synthesizing massive, disparate information sources.”44 Nonetheless, no matter whether a top-down or a bottom-up approach is adopted, it is humans who train AI/ML systems.
If the “orientation” phase ends with defining a number of courses of action (COAs), the next phase is when these COAs are compared and weighted to make the decision. Assuming, as a hypothesis, the complete reliability of AI systems, they could decide what option humans should implement. Time has proven to be a critical resource for success, and in the near future it looks as if every fraction of a second could be decisive. Humans are not likely to be self-sufficient in managing situations at the same pace AI/ML can do.45 Improved autonomous systems trained to implement fuzzy logic can provide accurate and fast decisions.46
During the “action” phase, AI can improve force protection. Indeed, AI/ML systems have the capability to run robotics and autonomous systems (RAS). Many different typologies of RAS are being tested to decrease the human involvement in combat and improve the performance of armed forces. Being able to count on advanced autonomy allows for RAS to be able to perform dangerous tasks for longer times and at greater distances while reducing the number of humans at risk.47
Balancing the Technological, Ethical, and Cultural Factors
In the future application of technological innovations, it is crucial to identify an intermediate point between what appear to be two extreme approaches humans have to AI. On the one side is the absolute skepticism and on the opposite side an unconditional trust to the use of AI. In the authors’ view, three factors should be considered to identify such an intermediate point: technological, ethical, and cultural.48 The position of this intermediate point, which might actually be either located at times closer to one side than the other, depends on the relevance of each of the three factors.
Figure 1. Balance of factors
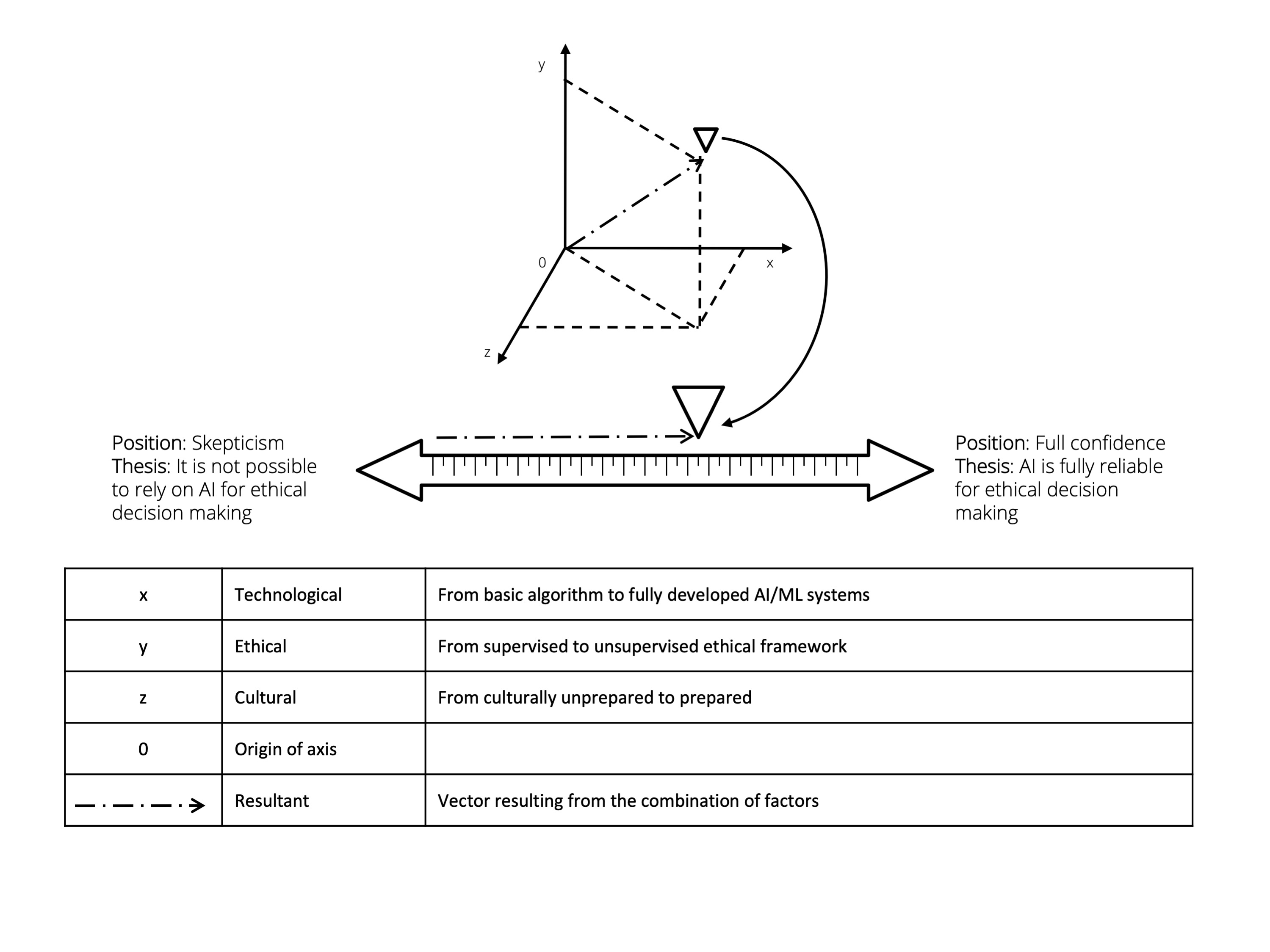
Source: courtesy of the authors, adapted by MCUP.
In figure 1, at the point of origin of the axes, the three factors are at their respective minimums. The minimum value represents a condition in which technology does not support data processing; ethics cannot be integrated into decision making; and, from a cultural point of view, it is not possible to accept that a machine can make decisions on behalf of human beings. The triangle represents the point at which the three factors reach the balance, creating the condition for an AI system suitable for effective and ethical decision making. The dotted-line arrow represents the distance from the origin to the balancing point.
Even though currently this balancing point has already moved away from the origin, AI is far from being a fully trustable support for decision making that has an ethical dimension. It is possible to describe the current situation using again the example of the GPS navigator. The technology allows processing of relevant data to identify the position in the world of an individual, relate it to a different geographical point, and evaluate all the variables (time, space, and laws) to provide such individual with the best path to reach the endpoint. The moral implications of this choice are simple enough that it is possible to make this decision ethically acceptable through a basic utilitarian model that maximizes the subject’s happiness while decreasing their suffering.
If the priority of the subject is the duration of travel, the AI will develop a path that although it is a longer route perhaps requiring some tolls, it is still the fastest compared to other options. In addition, applications like Google Maps are implementing new features to calculate routes that preserve gasoline consumption to help reduce CO2 emissions. Finally, human beings are now accustomed to using GPS and have embraced a culture that easily accepts such a tool to support decision making. It is also possible to conclude that humans trust GPS navigators because the three factors of technology, ethics, and culture blend together in a well-balanced, mutually supporting interaction. This example shows that humans trust GPS because they are used to its AI (cultural factor) that does the math right (technological factor) without incurring the risk of being immoral (ethical factor).
However, if one or more of these factors is off-balance, it would not be safe for humans to rely on AI systems. For example, it is interesting to imagine what might happen when one of the three factors compromise the overall balance. ML technologies might improve decision making, allowing a qualitative leap forward for humankind. Yet, due to the inherent design of its hardware and software, such a technology might be affected by a lack of transparency that could affect how humans control AI/ML systems.49
The potential lack of transparency should be offset; first by the possibility of making machine’s decisions morally acceptable through an ethical framework suitable for meeting the specific requirements for the task; second by an improved habit of using this technology by humans. The first mitigation avoids morally unwanted second- and third-order effects, while the second reduces humans’ natural fear of the unknown. This latter aspect deserves some more explanations.
Human superiority in decision making still exists, but AI might still be extremely helpful in situations in which this superiority is not enough. For example, the ability to always see the big picture, combined with a solid ethical background, makes humans sharper in broad spectrum decision making. Nonetheless, AI’s ability to process a more significant amount of data per second could make AI decisive in narrow and particular situations. Humans will have to acknowledge that, under certain conditions, it is possible that the best of their decisions might be worse than the AI systems’ worst ones. Indeed, when the enemy launches a missile attack, an accurate but late human decision about a countermissile artillery reaction is more dangerous than a not wholly right yet on time AI decision. This allows at least for mitigating damages due to AI's speed of decision making. In the future, technological improvements will allow the design of increasingly refined AI systems able to make the same types of decisions as humans. However, humans will train these AI systems directly (top-down) or indirectly (bottom-up) according to their knowledge or through their experiences.50 It is reasonable to believe that, at the end of the training, AI systems will be able to replicate the dynamics of human reasoning very closely; such a reasoning hopefully will include ethical thinking and will have the same fallacies that ethical thinking has in human beings. Nonetheless, humans should make reliance on AI a part of their culture, in particular when situations require processing a disproportionate amount of data in a very limited amount of time. Therefore, it is highly probable that AI systems will still make mistakes, yet given certain conditions (e.g., time available and amount of data), they could be more reliable than humans.
Machines’ fallibility might not be a problem, yet humans could hardly accept it. The problem is that, in some situations, especially those that involve people’s safety, the same mistake might be more tolerable if made by humans than by a machine. There are two reasons behind this distrust toward AI systems: first, it is accepted that humans can make mistakes while a machine should be flawless; second, there would be nobody to blame when an AI system gets it wrong. Indeed, it is possible to punish a human that has made a mistake, but not a machine.51
While these two reasons make it difficult to accept a machine’s decision about the safety of human beings, the perceived necessity of a decision-making support tool is at the base of the cultural propensity of humans to accept that an AI system might decide entirely or partially on their behalf.
Humans are committed to research and develop new technologies because they believe that such technologies will improve the well-being of humanity and people’s quality of life. This perception affects how much humans are willing to rely on AI. The more difficult it is for human beings to guarantee high standards of speed and effectiveness in a given task, the more they will feel the need for technological support in order to increase their performance and, as a result, they will be more willing to rely on machines. Therefore, humans would safely rely on AI systems as long as they see the machine’s worst performance as a better output compared to the human’s best performance on the same action.
Conclusion
Having seen the potential that AI has to improve humans’ efficiency in ethical decision making, it is crucial for individuals to make every effort to define objective parameters to identify an AI system’s balancing point. AI systems should be cataloged and associated with certain situational conditions (e.g., urgency, or the amount of information to be processed) to allow users to identify which ones bring the best benefit to their purpose.
In this way, military commanders could be better positioned to decide which tools to use and under what circumstances. Commanders can drastically reduce the time invested in decision-making processes and be aware of the incomplete suitability of a given system and to implement the necessary arrangements to mitigate the effects of possible errors. The importance of this process lies in the fact that AI is already widespread and accessible to all competitors. Therefore, not being able to optimize the use of AI systems would mean starting with a considerable disadvantage that could compromise the ability to achieve and maintain the initiative on the enemy, thus accepting fighting on the enemy’s terms. Very likely the employment of AI in the military decision-making process is unavoidable, and for this reason military leaders and AI developers might study how to build ethics into AI systems. There are different degrees of possible moral machines, from the implementation of basic utilitarian frameworks, up to ethically more complex and sophisticated systems. These different kinds of machines will be able to perform at different complex stages of the decision-making process.
Military leaders should be accountable for the decision they make. This accountability must also endure when AI systems are used to support their
decision-making process. Having a catalog that identifies what device is suitable and for what purpose in different situations is a fundamental condition to apportion responsibility on the right individual. If commanders intentionally do not use the appropriate device for a given mission, they are responsible for the decision. Yet, if commanders choose the correct device but the device fails, and if the follow-on investigation on other actors’ responsibility (e.g., AI designers, code developers) determines that none of the actors has a direct responsibility, probably humans should accept that the outcome was unpredictable.
Future studies should investigate how to assign a value to the weight of the three factors at the balance point. As far as technology is concerned, it could be a simple but effective way to rely on the possession or not of specific technical characteristics or certain components. Regarding ethics, it could be helpful to define a scale of values to be associated with a particular ethical model that is purely based on utilitarian logic or can also consider more profound implications or evaluate second- and third-order effects. Finally, the cultural factor could represent the most challenging obstacle to overcome due to its subjective and, in a certain sense, ephemeral nature. However, parameters such as the diffusion among the population or how long a device has been in use can be the starting points to establish values.
Endnotes
- The use of unsupervised autonomous weapons is growing fast and their use has proved to be an advantage. The case of the 2020 Nagorno-Karabakh war during which Azerbaijan used with excellent results the Israeli-made IAI HAROP Loitering Munitions against Armenia is evidence that such weapons can be extremely powerful. See Brennan Deveraux, “Loitering Munitions in Ukraine and Beyond,” War on the Rocks, 22 April 2022.
- Ann-Katrien Oimann, “The Responsibility Gap and LAWS: A Critical Mapping of the Debate,” Philosophy & Technology 36, no. 3 (2023): 7–16, https://doi.org/10.1007/s13347-022-00602-7.
- ML does not preclude developing a sense of curiosity. Yet, today AI/ML systems are not able to learn something if humans do not direct them to do so. For example, a thermostat based on an AI/ML system can learn how to improve comfort for humans in their houses, but it is not curious to learn about how to play chess until a human changes its algorithm.
- Ragnar Fjelland, “Why General Artificial Intelligence Will Not Be Realized,” Humanities & Social Sciences Communications 7, no. 1 (2020): 2, https://doi.org/10.1057/s41599-020-0494-4.
- Fjelland, “Why General Artificial Intelligence Will Not be Realized,” 7.
- James E. Baker, The Centaur’s Dilemma: National Security Law for the Coming AI Revolution (Washington, DC: Brookings Institution Press, 2020), 14.
- Brian David Johnson, “Autonomous Sentient Technologies and the Future of Ethical Business,” in Ethics @ Work: Dilemmas of the Near Future and How Your Organization Can Solve Them, ed. Kris Østergaard (Middletown, DE: Rehumanize Publishing, 2022), 69.
- Stuart J. Russell and Peter Norvig, Artificial Intelligence: A Modern Approach (Hoboken, NJ: Pearson, 2009), 2–5.
- The authors agree with the fact that a strong Kantian view of moral behavior applied to human beings is implausible. Here, we focus on machines whose behavior is determined by the specific input they have received. As Russel and Norvig suggest, machines think rationally and act rationally. To think like humans and behave like humans, they will need to use ML and adopt a nonbinary logic approach.
- Artificial neural networks (ANNs) are among the most used solution to implement learning skills on machines. The main idea of this technology is to mimic the human brain’s neural connections throughout different layers of artificial neural nodes. Incorrect outputs generate adaptation in the inner and hidden neural layers until the results are correct. Therefore, machines that are more experienced would be more effective. Yet, this technology still suffers transparency issues because, like a human brain, it is not clear how machines manage these adaptations. This lack of transparency could likely prevent human understanding if and why failures occur in case of false positive output.
- Bahman Zohuri and Moghaddam Masoud, Neural Network Driven Artificial Intelligence (Hauppauge, NY: Nova Science Publishers, 2017), 15–17.
- James H. Moor, “The Nature, Importance, and Difficulty of Machine Ethics,” IEEE Intelligent Systems 21, no. 4 (2006): 19–20, https://doi.org/10.1109/MIS.2006.80.
- Moor, “The Nature, Importance, and Difficulty of Machine Ethics,” 20.
- Moor, “The Nature, Importance, and Difficulty of Machine Ethics,” 20.
- Vincent Conitzer et al., “Moral Decision Making Frameworks for Artificial Intelligence,” Proceedings of the AAAI Conference on Artificial Intelligence 31, no. 1 (2017): 1–5, https://doi.org/10.1609/aaai.v31i1.11140.
- Conitzer et al., “Moral Decision Making Frameworks for Artificial Intelligence,” 1–5.
- Noah J. Goodall, “Ethical Decision Making during Automated Vehicle Crashes,” Transportation Research Record 2,424, no. 1 (2014): 7–12, https://doi.org/10.3141/2424-07.
- Goodall, “Ethical Decision Making during Automated Vehicle Crashes,” 7–12.
- Goodall, “Ethical Decision Making during Automated Vehicle Crashes,” 7–12. What happens in the hidden layers of ANN is obscure to humans. Software developers are not able to understand how ANN-based machines reach their output and how they correct themselves during the process. An interface that translates machine language into a natural language would be beneficial in understanding how machines work and where and why possible mistakes occur.
- Goodall, “Ethical Decision Making during Automated Vehicle Crashes”; and Alan F. Winfield and Marina Jirotka, “Ethical Governance Is Essential to Building Trust in Robotics and Artificial Intelligence Systems,” Philosophical Transactions of the Royal Society A: Mathematical, Physical and Engineering Sciences 376, no. 2,133 (2018): https://doi.org/10.1098/rsta.2018.0085
- Conitzer et al., “Moral Decision Making Frameworks for Artificial Intelligence,” 1–5; and Goodall, “Ethical Decision Making during Automated Vehicle Crashes.”
- Amitai Etzioni and Oren Etzioni, “Incorporating Ethics into Artificial Intelligence,” Journal of Ethics 21, no. 4 (2017): 403–18, https://doi.org/10.1007/s10892-017-9252-2.
- Etzioni and Etzioni, “Incorporating Ethics into Artificial Intelligence,” 406–7.
- Etzioni and Etzioni, “Incorporating Ethics into Artificial Intelligence,” 405–6.
- Zohuri and Masoud, Neural Network Driven Artificial Intelligence, 15–17; and Etzioni and Etzioni, “Incorporating Ethics into Artificial Intelligence,” 403–18.
- Etzioni and Etzioni, “Incorporating Ethics into Artificial Intelligence,” 403–18.
- Etzioni and Etzioni, “Incorporating Ethics into Artificial Intelligence,” 406–7.
- Etzioni and Etzioni, “Incorporating Ethics into Artificial Intelligence,” 407.
- Forrest E. Morgan et al., Military Applications of Artificial Intelligence: Ethical Concerns in an Uncertain World (Santa Monica, CA: Rand, 2020), xiii–xiv, https://doi.org/10.7249/RR3139-1.
- Mark Ryan, “In AI We Trust: Ethics, Artificial Intelligence, and Reliability,” Science and Engineering Ethics 26, no. 5 (October 2020): 13–14, 2,749–67, https://doi.org/10.1007/s11948-020-00228-y.
- Ross W. Bellaby, “Can AI Weapons Make Ethical Decisions?,” Criminal Justice Ethics 40, no. 2 (2021): 86–107, https://doi.org/10.1080/0731129X.2021.1951459.
- Bellaby, “Can AI Weapons Make Ethical Decisions?,” 95–97.
- Morgan et al., Military Applications of Artificial Intelligence.
- Anupam Tiwari and Adarsh Tiwari, “Automation in Decision OODA: Loop, Spiral or Fractal,” i-manager’s Journal on Communication Engineering and Systems 9, no. 2 (2020): 1–8, http://dx.doi.org/10.26634/jcs.9.2.18116.
- Tiwari and Tiwari, “Automation in Decision OODA,” 16–17.
- Etzioni and Etzioni, “Incorporating Ethics into Artificial Intelligence.”
- AI-ML’s computing rate is growing constantly, not considering quantum computing. For this reason, the expectation that AI will be able to process large amounts of information is realistic. If the information—any amount of information—fed to the algorithms is accurate and has the correct standard, AI will continue to provide increasingly better outcomes. The problem is when a large amount of information is provided to the AI unfiltered and without a proper application of correct standard.
- Daniels J. Owen, “Speeding Up the OODA Loop with AI: A Helpful or Limiting Framework” (paper, Joint Air & Space Power Conference, 7–9 September 2021), 159–67.
- Owen, “Speeding Up the OODA Loop with AI,” 159–67; and Baker, The Centaur’s Dilemma, 30–31.
- The U.S. Army Robotic and Autonomous System Strategy (Fort Eustis, VA: U.S. Army Training and Doctrine Command, 2017), 10.
- Tiwari and Tiwari, “Automation in Decision OODA,” 1–8.
- Fernando De la Cruz Caravaca, “Dynamic C2 Synchronized Across Domains: Senior Leader Perspective” (paper, Joint Air & Space Power Conference, 7–9 September 2021), 81–89.
- Tiwari and Tiwari, “Automation in Decision OODA,” 1–8.
- Owen, “Speeding Up the OODA Loop with AI,” 159–67.
- Owen, “Speeding Up the OODA Loop with AI,” 159–67.
- Michael Doumpos and Evangelos Grigoroudis, eds., Multicriteria Decision Aid and Artificial Intelligence: Links, Theory and Applications (Chichester, UK: John Wiley & Sons, 2013), 34.
- The U.S. Army Robotic and Autonomous System Strategy, 3.
- This idea does not necessarily depart from the majority of the literature. This idea strives to provide a better approach on how to assign responsibility when using AI in the decision-making process. Defining parameters that clearly put in relation each AI system with its possible field of employment would be beneficial. For example, defining responsibility for choosing the right AI system for the specific situation. This choice should not be linked only to the question: Is this system able to make this decision? It should comprehend also questions related to the ability to be ethical in the decision-making process and to the humans’ acceptance rate for that specific AI system.
- Alan F. Winfield and Marina Jirotka, “Ethical Governance Is Essential to Building Trust in Robotics and Artificial Intelligence Systems,” Philosophical Transactions of the Royal Society A: Mathematical, Physical and Engineering Sciences 376, no. 2,133 (2018): 6, https://doi.org/10.1098/rsta.2018.0085.
- Etzioni and Etzioni, “Incorporating Ethics into Artificial Intelligence,” 403–18.
- Alexander McNamara, “Majority of Public Believe ‘AI Should Not Make Any Mistakes’,” Science Focus, 6 July 2020.