David E. McCullin, DM
31 March 2023
https://doi.org/10.36304/ExpwMCUP.2023.04
PRINTER FRIENDLY PDF
EPUB
AUDIOBOOK
Abstract: This article offers a literature review that results in the introduction of three information literacy protocols. It is the third in a four-part series of articles that discusses the integration of the evidence-based framework (EBF) and military judgment and decision making (MJDM). The series is written as a conceptualization and implementation of the U.S. presidential memorandum on restoring faith in government dated 27 January 2021. The memorandum directs federal agencies to base policy- and decision-making processes on the best available evidence. Accordingly, the focus of the series is on integrating the EBF into defense planning and decision making as an operational art. This article focuses on information literacy—specifically, building a data set for systematic reviews. The current literature on information literacy reveals that specific protocols to enhance rigor, transparency, validity, and reliability in building a data set for systematic reviews are significantly underrepresented. In this article, the Stanford Design Thinking Process model was applied to address this underrepresentation. The Stanford model uses five phases of collaboration to develop new protocols. The results of these collaboration are three new protocols for building data sets that are specifically designed for an integration of the EBF and MJDM: the targeting search strategy protocol (TSSP); the research question variable synthesis protocol (RQVSP); and the human source query protocol (HSQP).
Keywords: information literacy, evidence-based framework, evidence-based management, EBM, military planning, military judgment and decision making, MJDM, systematic review, design thinking
The first article in this series presented research that suggested that an integration of the evidence-based framework (EBF) and military judgment and decision making (MJDM) is feasible. The research was presented in a critically appraised topic (CAT) using systematic review methodologies.1 The second article focused on how this integration could occur at the Joint planning level.2 This article focuses on the importance of building a data set for systematic reviews. The final article in this series will explore evaluating information for systematic reviews.
Figure 1. Evidence-based framework
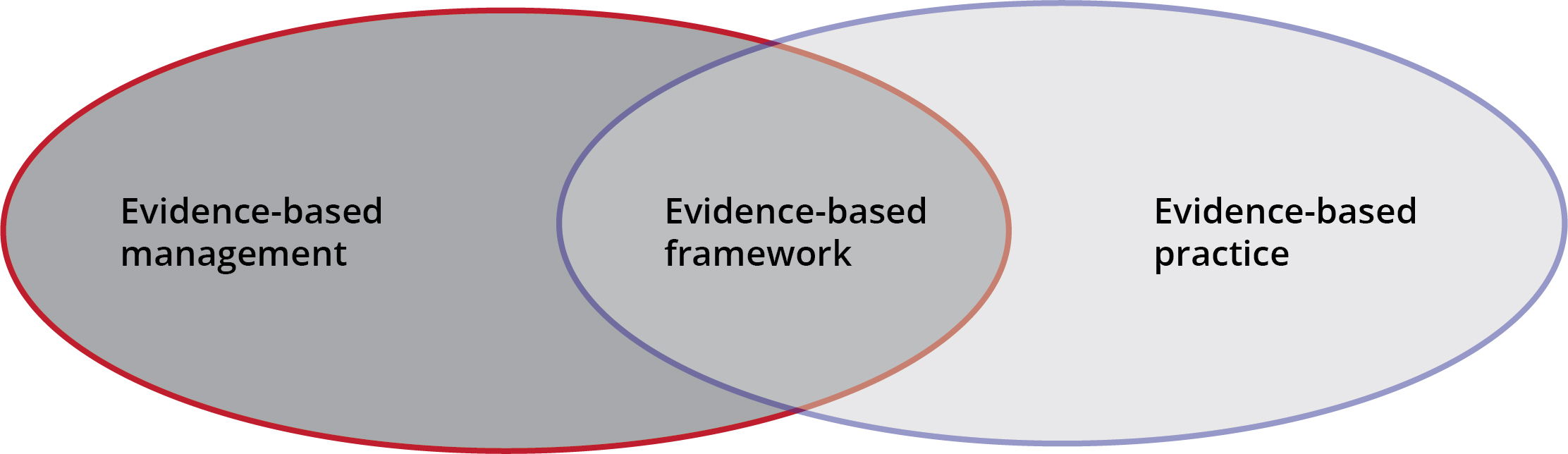
Source: courtesy of the author, adapted by MCUP.
Evidence-based management (EBM) creates evidence with a framework of asking, acquiring, appraising, aggregating, applying, and assessing.3 These six phases are made transparent by the systematic review, which details the judicious use of information in a rigorous format that is specifically designed for social science research. As evident by its name, the systematic review is designed to methodically identify trends within large data sets through analysis and synthesis.
Definitions
The following definitions are provided here to offer context and clarity to the terms used in this article. They represent a compilation of evidence and experiences.
- Information literacy: the set of integrated abilities encompassing the reflective discovery of information, the understanding of how information is produced and valued, and the use of information in creating new knowledge.4
- Data set: a collection of data related to a specific path of inquiry.
- Evidence-based management (EBM): a decision-making framework that draws evidence from experience, stakeholder input, organizational data, and scholarship.5
- Evidence-based practice (EBP): the employment of a methodology of asking, acquiring, appraising, aggregating, applying, and assessing.
- Evidence-based framework (EBF): the integration of EBM and the EBF, as executed in a systematic review.6
- Human sources: stakeholder input and subject matter expertise within the EBF.7
- Interface: the mechanism employed by a researcher to locate and extract data from its source.8
- Military judgment and decision making (MJDM): a spectrum of decision-making processes related to the arts and sciences of national defense. Within this spectrum, quantitative and qualitative processes are used to make decisions based on multiple courses of action.
- Research framework logic: a portfolio of frameworks from which authors choose that define specific parameters to guide the development of a research question and validate data set content. A common thread within the portfolio is the use of variables that define who the study impacts, the instrument(s) used, and what is expected or what the study will produce.9
- Research question: a question developed from a pending decision to focus a research effort that is designed to create evidence.10
- Stakeholders: individuals or organizations directly impacted by a judgment or decision.11
- Systematic review: a method of social science research that follows the scientific method. Systematic reviews explore relationships between variables to address hypothetical research questions.12
- Targeting: considering an entity or object for possible engagement or other action. Targeting requires a decision to engage, detection of the entity or object, delivery of a sortie, and assessment of the impact.13
Literature Review
The literature on building data sets for systematic reviews identifies clear trends regarding scholar practitioners, databases, and search engines. However, the literature shows no clear trends in identifying protocols for locating the best available information from human and institutional sources. Today’s scholar practitioners are specifically challenged by an abundance of information, which creates constraints in building data sets. The diversity and accessibility of human knowledge, multidisciplinary search engines, and public and private domains are among the constraints practitioners face when building data sets. Even with the presence of these constraints, the literature does not define specific search protocols for systematic reviews.
Both multidisciplinary and subject-specific search engines are identified in the literature by name and categorized by the content within their associated databases. Search engines such as Google Scholar, Microsoft Academic, and Bielefeld Academic Search Engine (BASE) are cited in the literature as multidisciplinary because they contain general scholarship. There are search engines for domain-specific bibliographic information, such as the DBLP computer science bibliography for computer science scholarship, PubMed and GoPubMed for biomedical literature, and the arXiv repository for physics, mathematics, and computer science. There are also digital libraries with online public access catalogs that are dedicated interfaces for domain-specific research. The literature shows that scholar practitioners fuse constructs such as heuristics, domain knowledge, taxonomies, thesauri, structures, and word relationships to improve search quality.14 Although these digital interfaces and practitioner rolls are identified in the literature, specific protocols for optimizing the quality of the search are not.
Databases
Dion Hoe-Lian Goh et al. describe how data search and retrieval methods have changed the way people access and interact with information. They also explain how innovations in these areas have extended the concept of libraries far beyond physical boundaries. Database availability through personal computers has shifted many of the functions of librarians to researchers, thereby extending the library beyond its traditional physical boundaries.15
Muhammad Rafi, Zheng Jian Ming, and Khurshid Ahmad posit that in the past, a lack of current literature and database resources slowed scholars’ scientific research. Since the 1990s, however, technology has rapidly evolved. The internet has created the opportunity for libraries to shift subscriptions from academic journals to databases with access to thousands of journals and electronic books. These online database resources deepen the availability of electronic resources and extend the boundaries of academic research.16
Krutarth Patel et al. describe how online scholarly digital libraries usually contain millions of scientific documents. For example, Google Scholar is estimated to have more than 160 million documents. Open-access digital libraries have witnessed a rapid growth in their collections as well. For example, CiteSeerX’s collections increased from 1.4 million documents to more than 10 million within the last decade. These rapidly growing scholarly document collections offer rich domains of specific information for knowledge discovery, but they also pose many challenges to the navigation of and search for useful information.17
Paula Younger and Kate Boddy describe how the vast amount of databases associated with a single interface can deliver duplicate studies. Because there are a wide variety of databases and associated interfaces in existence, it is important to concentrate on interface inputs and resulting outputs, the goal being to achieve consistent outputs regardless of the database or interface. To optimize this effect, the search strategy must account for using a single search string across multiple interfaces with multiple differing databases.18
Shaul Dar et al. highlight the vast quantity of data that is warehoused in corporate and academic databases. This information is often not accessible because the database proprietor may have established complex user interfaces that require knowledge of programming languages to retrieve the data. Overall, research can be enhanced by an intuitive user interface that impacts multiple databases.19
Scholar Practitioners
Maureen O. Meade and W. Scott Richardson explain the roll of the scholar practitioner in selecting studies for systematic review. Although there are no specific protocols, this is typically done through the application of established strategies. Scholar practitioners are obliged to ensure that the strategies they use afford rigor and transparency within all studies they select.20 These include peer review, screening criteria, and elimination strategies. The practitioner should predetermine screening criteria for the studies to be included in the systematic review. They should also identify elimination strategies that are applied during appraising phase of the systematic review.
Sherry E. Mead et al. conducted studies examining the influences of general computer experience and age on library database search performance among novice users. They found that computer experience offers a slight advantage over age among novice users in search tasks. However, age closed the performance gap in search success, syntax errors, database field specifications, keyword specifications, and use of Boolean operators. Although age was a minor factor, novice users with general computer experience correlated with better performance.21 As mentioned earlier in this article, researchers have taken on some of the functions of librarians due to the prevalence of and access to online databases.
Although researchers have indeed taken on some roles historically restricted to librarians, Rosalind F. Dudden and Shandra L. Protzko’s research on the work of librarians on a systematic review demonstrates that librarians can play an important role in the systematic review process. The authors found that librarians possess valuable expertise in critical areas of developing search strategies, and that they add value as organizers and analyzers of the search results.22 Similarly, Susan A. Murphy and Catherine Boden explore the participation of Canadian health sciences librarians on systematic reviews. Their study seeks to create a benchmark role for librarians in systematic reviews. These two studies reveal that although librarians maintain traditional roles, they can also make valuable contributions as a systematic review team member and/or scholar practitioner.23
Aldemar Araujo Castro, Otávio Augusto Câmara Clark, and Álvaro Nagib Atallah conducted a meta-analysis study to discover an optimal search strategy for clinical trials in Latin American and Caribbean electronic health science literature databases. The study combined headings with text in three languages while adapting the interface and identified search terms of high, medium, and low precision, where precision was demonstrated by the articles returned from a search terms.24 In terms of scholar practitioner effort, this suggests that time spent on optimizing the search string will likely improve the data set.
Search Engines
Regarding search engines, Wichor M. Bramer et al. posit that scholar practitioners searching for studies for systematic reviews will typically interface one search engine that will query multiple databases simultaneously, causing duplicate studies to appear in the search results. This study shows that a practical and efficient method for reducing duplication resulting from a single search engine accessing multiple databases is lacking.25 After comparing Google internet search engines with academic library search engines, Jan Brophy and David Bawden found that “Google is superior for coverage and accessibility while library systems are superior for quality of results. Precision is similar for both systems. Good coverage requires use of both, as both have many unique items. In addition, he concluded that improving the skills of the searcher is likely to give better results from the library systems, but not from Google.”26
According to Lov K. Grover, one problem related to data searching occurs during the screening and sorting processes. When one source within a database satisfies a given search parameter, it must be further screened by reading through the abstract and key terms. This screening and sorting strategy determines if the search parameters were satisfied. This is a default strategy of scholar practitioners because most search interfaces are not automated beyond returning search results. Because of this interface algorithm, multiple searches will be required before finding the desired data set.27
Jody Condit Fagan’s study of usability testing of a large, multidisciplinary library database illustrates three distinct factors. First, search strings with Boolean connectors are still the most prevalent method of retrieval. Second, library products often have more metadata and organizational structure than web search interfaces that are adapted for scholarly research. Third, graphic interfaces offer great benefit to the user, emphasizing the faster perceptual processes of pattern recognition.28
Bramer et al. state that digital object identifiers (DOIs) are unique tags for locating source articles within academic journals. Because they are unique, they could be used for deduplication. However, the article reveals that DOIs are not present in every database. When they are present, they often cannot be easily exported with the source article. Consequently, DOIs cannot be relied on to identify duplicates. Their alternative involves using pagination, due to the large numbers of pages in scientific journals. In combination with other fields, pagination can serve as a type of unique identifier for deduplication.29
This literature review highlights the difficulty scholar practitioners face in integrating different databases and search engines to build data sets for systematic reviews. Daniel Hienert et al. prescribe open-source technology, established metadata schemes, and terminology mappings between different thesauri to overcome some of the typical retrieval problems. The challenge for the practitioner in the search is to create data sets.30 The following section adds context to the relationship between the EBF, the systematic review, and the importance of building a data set.
This article draws from four themes within the panacea of literature on creating data sets for systematic reviews: the database; the search engine; the scholar practitioner; and a lack of search optimization protocols. The database theme provides context to conceptualize the idea that the emergence of electronic storage of scholarship has ultimately resulted in the emergence of the EBF. The search engine theme provides context for a requirement to have a rigorous process in place to search for the best evidence from all sources. The scholar practitioner theme provides context for a professional, ethical, and efficient effort in building a data set. The protocols introduced later in this text provide guidance for optimizing the quality of the search.
The Evidence-Based Framework and Search Strategy
The EBF makes judicious use of information that is extracted from data sets specifically built to address a particular path of inquiry. The systematic review guides and documents the EBF. Because each data set is specifically created to address a specific path of inquiry, the process of creating a data set is a critical component of the EBF’s validity and reliability. This means that the quality of the data set is a function of the data it contains. The single determinant that ensures quality in any data set is information literacy. The format that makes information literacy transparent is the systematic review, which contains a specific section for the search strategy.
A data set is created from human and institutional sources. Human informational sources provide subject matter expertise and stakeholder input while institutional sources provide scientific studies and organizational data. To implement the EBF, scholar practitioners balance the integration of human and institutional sources in creating data sets. According to Eric Berends, Denise M. Rosseau, and Rob B. Briner, the best available evidence—as well as the perspectives of those people who might be affected by the decision—epitomizes the core concept of EBM.31
Although this article focuses on the acquiring phase of the systematic review, the constructs of the asking phase are also integrated here. Asking is the process of formulating a specific question, which allows evidence to be compiled that will inform a decision. Once a question is formulated, the acquiring phase begins. In this phase, acquisition commences with a search for both human and institutional data. The acquisition culminates with a data set that is structured for an appraisal.
The search strategy is an important part of the systematic review because it supports rigor and transparency. Therefore, its importance should not be minimized. As discussed, the systematic review is the tool commonly relied on to pose questions, guide the research process, and present findings. The validity and reliability of the systematic review is supported by evidence that comes from building a data set of the best available information. A common axiom associated with the systematic review is “no evidence, no review.”32 Based on this axiom, one can conclude that the search is the key measure of rigor and transparency of the systematic review because the search is how a data set is built. This conclusion is a key theme of this article. The processes used to acquire a systematic review data set should be as rigorous as possible and recorded in detail.
Design Thinking and Data Set Building
The Stanford Design Thinking Process, introduced at the Hasso Plattner Institute of Design (d.school) at Stanford University in 2005, has been applied to developing prototypes for improving information literacy.33 A design team of subject matter experts in military judgement and decision making, military intelligence, and the EBF have collaborated in this effort. In the following section, design thinking and the results of this collaboration are explained.
Design thinking is a mindful, human-centered, action-oriented approach to problem solving that develops prototypes from radical collaboration.34 The Stanford model employs a six-step process, as shown in figure 2. The scope of the Stanford model’s application in this article was limited to the first four steps of the Design Thinking Process: empathize; define; ideate; and prototype.
Figure 2. Stanford Design Thinking Process
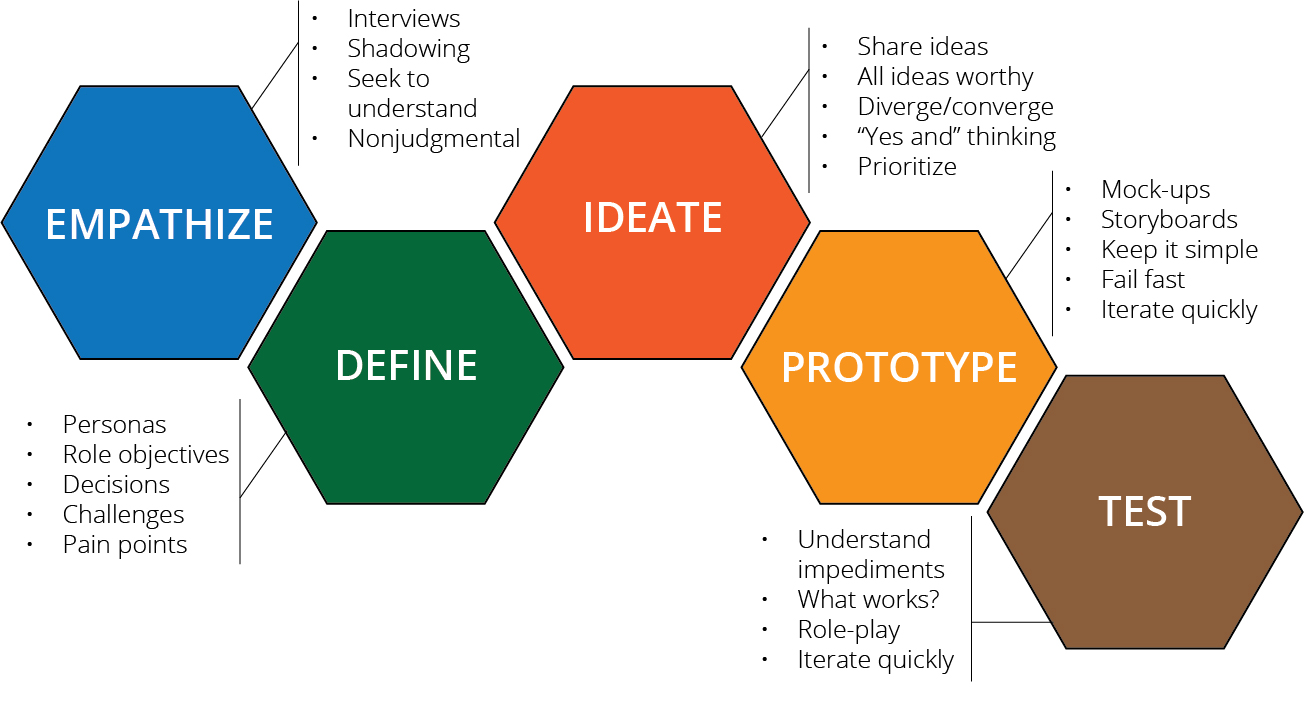
Source: courtesy of Stanford d.school, adapted by MCUP.
Design Thinking Applied
Empathize
Empathy in design thinking is a matter of perspective.35 The thought process used by the design team in this conceptualization exercise was, to put simply, “walking a mile in the stakeholders’ shoes.” In this case, the stakeholders are the would-be planning practitioners executing the EBF in MJDM processes. From this perspective, the determinants of success are a strategic approach that provides the scope for building data sets as well as clearly defined steps to follow that include interfaces to incorporate data from both human and institutional sources. The perspective gained from the empathy of walking a mile in the stakeholders’ shoes provides an understanding of the variables encountered from interactions within a defined problem space.
Define
In the Stanford Design Thinking Model, the process of defining frames the problem with perspectives gained from empathizing.36 For example, the process of empathizing allowed the design team to identify determinants of success to build an EBF construct in a MJDM process. In this case, the problem of building data sets was framed in terms of rigor, transparency, validity, and reliability. These concepts are accepted measures for mitigating the biases associated with bounded rationality, which can be defined as the limited capacity of human cognition in acquiring and processing information.37
Ideate
Ideating focuses on idea generation.38 The design team initiated the thinking process with a brainstorming session, in which the application of context (provided by empathizing and defining) and generation of solution-oriented ideas were generated through collaboration. The session focused on a theme of rigor, transparency, validity, and reliability in the search process. The session began by referencing the literature review herein.
The literature on the search process revealed three common threads, which are discussed below. From these areas, it is possible to synthesize some general best practices on building data sets. However, the literature shows gaps in introducing a comprehensive search model that introduces rigor, transparency, validity, and reliability to the search process. An additional gap in the available literature identified a lack of scholarship on interfacing with human data sources to integrate stakeholder and subject matter expertise into building data sets. Creating such a methodology was the focus of the design team’s ideation.
Solutions for a rigorous, valid, transparent, and reliable search process centered on three areas. First, the search process would build data sets for systematic reviews that will be embedded in MJDM. Therefore, referencing a military process for searching and identifying targets would facilitate understanding and emphasize the importance of searching for and identifying literature for a data set. Second, creating rigorous, transparent, valid, and reliable search models contributes to the body of knowledge on building data sets and has immediate practical application to an integration of EBM and MJDM. Third, creating a process for querying human sources would also contribute to the body of knowledge on building data sets for systematic reviews with immediate practical application to an integration of EBM and MJDM.
Prototype
The prototype model is the iterative generation of protocols intended to bring one closer to a final solution.39 The questions to be addressed by the ensuing prototypes were as follows:
- What are the existing MJDM protocols that will help facilitate integration with the EBF?
- What specific steps in a search process would ensure rigor, transparence, validity, and reliability?
- How can human source data be integrated into the EBF and systematic review?
The design thinking application produced three prototypes, each with their own execution protocols related to creating a data set: the targeting search strategy protocol (TSSP); the research question variable synthesis protocol (RQVSP); and the human source query protocol (HSQP).
Test
Testing involves generating data to refine the prototype and assessing the results. This process may lead back to ideation if necessary and continue to be refined until a final prototype is developed.40 In this case, testing will be left to the scholar practitioners who are responsible for executing this process. Specific recommendations were made in the first two articles in this series. The first article recommended how to educate scholar practitioners for this integrated process.41 The second article spelled out how this integration could be executed within the confines of Joint Planning, Joint Publication (JP) 5-0.42 Testing should occur as the process is executed as recommended.
Building Data Sets
As discussed in the previous article in this series, the practice of creating evidence for decision making leverages the vast databases of social science research made possible by digital technology. These databases warehouse hundreds of thousands of studies in academic journals.43 In a systematic review, a data set is built by searching databases and journals for studies that address the research question. The data set is the crucial component of the systematic review, and the search is a crucial part of building a data set. The search strategy establishes the quality of the data set with inclusion and exclusion criteria.
The position of this article is that if the search demonstrates rigor and transparency, the subsequent data is more likely to be valid and reliable. Rigor is demonstrated by a strict adherence to a methodical process. Transparency is demonstrated by a detailed description of the process. The process should be documented with sufficient detail so as to provide a road map that allows it to be reliably replicated. In the systematic review, it is the analysis and synthesis of valid studies that contribute to a body of knowledge. The search is where the integration of rigor, transparency, validity, and reliability is initiated.
Within the context of this article, building a data set is viewed as an exercise of integrity in addition to rigor, transparency, validity, and reliability. Integrity in a data set is akin to integrity used in buying a home, buying a car, or online dating. In these three examples, an inquiry and a set of criteria are combined to inform a decision. The inquiry in these examples has to do with finding the right match within a large field of possibilities. The criteria in these examples have to do with narrowing a large field of choices down to a small field that represents the best available choices. The same logic used to buy a home, buy a car, or find a date online is the basis of integrity used in building a data set for a systematic review. In building a data set, integrity is not only logical but also critical to the process in three specific areas: interrogative integrity; practitioner integrity; and documentation integrity.
In the area of interrogative integrity, the quality of the data set is improved when the integrity between the research question and data is evident. In systematic reviews, evidence is created by extracting data from a primary source in which it has been documented or through interviews. Primary sources range from articles in peer-reviewed journals that describe hypothesis testing, to stakeholders and subject matter experts, to social media posts. The importance of interrogative integrity is matching the source material with the question one is attempting to answer.
In the area of practitioner integrity, a study is strengthened when the process demonstrates that the practitioner who is executing the study has taken measures to reduce bias in the search process. Therefore, practitioner integrity is manifested in rigor, as the practitioner systematically adheres to a preestablished search strategy. Practitioner integrity outlines a process for opening the data search to the widest possible sets of sources and narrowing the field of data to the most relevant sources.
In the area of documentation integrity, the study is strengthened by clear concise documentation of the search that will allow it to be replicated. Documentation integrity captures for record the methods of practitioner integrity. This includes bias mitigation strategies, which explain the disposition of outlier data as well as all data normalization processes employed. For example, during a typical search for systematic review data, the practitioner may encounter situations in which a syntax error in a search string impacts the search results or the initial search strings produce too few sources. In this case, documentation integrity illustrates how accurately the errors are documented by the practitioner.
Prototypes and Protocols
Targeting Search Strategy Protocol (TSSP)
In the design thinking application, the question guiding development of a search strategy prototype for an integration of the EBF and MJDM was as follows: What are the existing MJDM protocols that will help facilitate integration with the EBF? The TSSP is based on military targeting as described in Joint Targeting, JP 3-60. Within this Joint publication, there is a four-function targeting cycle that is commonly employed by land and maritime commanders. The functions are decide, detect, deliver, and assess.44 This section provides a brief description of each of the targeting functions and describes how it integrates in to the asking and acquiring phases of the EBF. Figure 3 shows the integration as described in this paragraph.
Figure 3. Integration of targeting and the evidence-based framework

Source: courtesy of the author, adapted by MCUP.
The Decide Function in the Evidence-Based Framework
The decide function establishes the strategy for detecting targets and identifies the delivery systems available. In the asking phase of the EBF, the decide function becomes the mechanism for establishing priorities to formulae the research question. In the acquiring phase of the EBF, the decide function becomes the protocol to set priorities for locating data, inclusion/exclusion criteria, and the type of interface used for data acquisition.
The Detect Function in the Evidence-Based Framework
The detect function gathers information about what the target is and where it can be located. The priorities set in the decide function provide the guidance to assign sorties in the deliver function.45 In the acquiring phase of the EBF, the detect function becomes the protocol to define and locate data sources and assign an interface for the data search.
The Deliver Function in the Evidence-Based Framework
In the deliver function, the main objective is to attack targets in accordance with the guidance provided by the decide function and identified in the detect function. Any sortie launched at a target is first defined and carefully coordinated prior to launch.46 In the acquiring phase of the EBF, the deliver function becomes the protocol for conducting search queries from human and institutional sources.
The Assess Function in the Evidence-Based Framework
In the assess function, the commander and staff assess the results of targeting. The assessment determines when the targeting objectives are met, and the process is repeated until that determination is made.47 In the EBF, the assess function becomes the protocol for refining search strings. For institutional data sources, this process is also repeated until the unified final search string is executed. For human sources, the query cycle also continues until they are refined into final recommendations.
These protocols for executing targeting functions in the EBF provide the guidance that the military scholar practitioner applies to building a data set. The protocols of the targeting functions and the asking and acquiring phases of the EBF create two adaptations to MJDM. First, their expression emphasizes the importance of the search. Second, they provide specific protocols that merge the functions of the military planner and the scholar practitioner, creating a role for the military scholar practitioner.
Human Source Query Protocol (HSQP)
The previous articles in this series detailed the importance of information taxonomy in identifying where to locate human versus institutional data.48 Because institutional data is archived, the search for this type of data will likely involve querying databases of scholarship or organizational servers. Human data, on the other hand, comes from stakeholders and subject matter experts and will therefore require structuring, as depicted in figure 4. The means of identifying human sources and obtaining meaningful data from them should be carefully planned and detailed in the search strategy.
Figure 4. Building human data sources
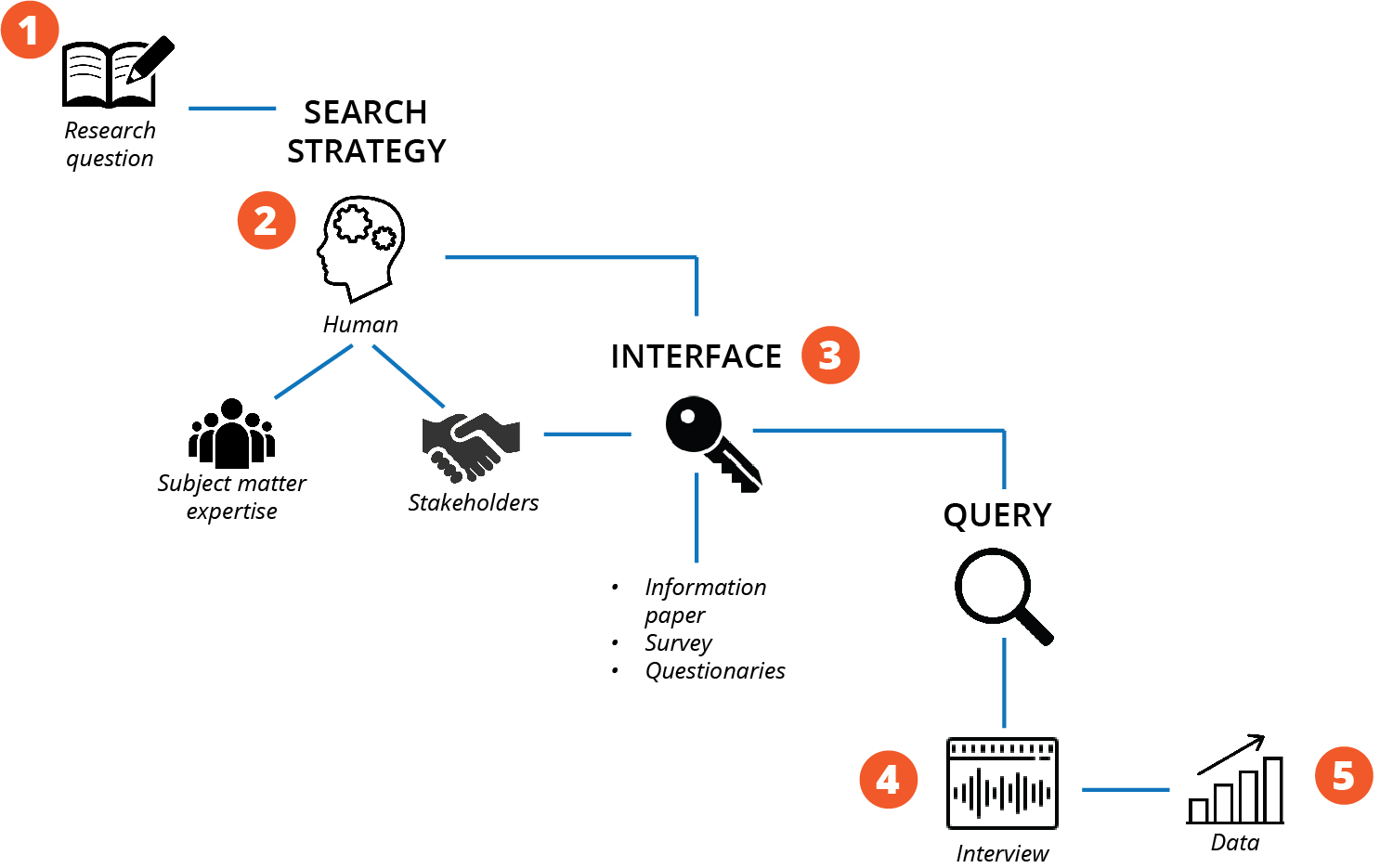
The protocols used here are as follows: 1) reference research question and search strategy; 2) locate information sources; 3) select appropriate interface tools; 4) conduct query; and 5) extract data.
Source: courtesy of the author, adapted by MCUP.
As indicated, the guiding principle behind any search strategy is finding the best available evidence. In institutional data searches, the population of data sources most often exceed the limits of human processing capacity and require computer processing to assist with the search. Similarly, each human source can be treated as a separate database in which the key to a successful query is in the interview strategy. A human search strategy should explain how interaction with the source focuses the information on the research question, the interface employed, and how the information is to be structured.
In the design thinking application, the question guiding development of a prototype for human data searches was as follows: How can human source data be integrated into the EBF and the systematic review?
As with institutional data, interfaces that screen, inform the source, focus the query, and reduce bias are a critical component of the search. Ultimately, a recommendation is needed from a human source. Therefore, screening any human sources is ultimately a question of acceptable bias. The scholar practitioner must pay particular attention to reducing bias in the interface tools. To interface with human sources, surveys and questionnaires can be designed to focus the query on the research question. In addition, there must be some interface that informs the human source of the problem area and the question guiding the research.
Screening human sources requires considerations to mitigate bias. Subject matter experts must consider the level and timeliness of experience and specific areas of expertise. Full transparency in the interfacing tools is essential to this end state. Although stakeholders offer uniquely valuable perspectives to any forthcoming decision-making process, they are also equally prone to bias as any other source. As with subject matter experts, the interface for screening stakeholders should also include their knowledge of the problem area and the research question. The practitioner should weight the acceptable level of bias among stakeholders and employ strategies to mitigate the risks associated with bias. The search strategy in the systematic review should include when and how stakeholder input will be integrated into the decision-making process, as well as when and how it will not be included. The inclusion and exclusion criteria should be clearly explained in the search strategy.
Research Question Variable Synthesis Protocol (RQVSP)
In the design thinking application, the question guiding development of a prototype for rigor and transparency in the search were as follows: What specific steps will ensure that rigor, transparency, validity, and reliability are embedded in the search process? The RQVSP has specific rules for creating search strings and narrowing the search until a unified final search string delivers the contribution to the final data set. Rigor is ensured by clear steps that define and refine the search process in order to create the unified final search string. These steps can be meticulously documented as a list of reporting items to provide the transparency necessary for replication.
The RQVSP model is presented and explained in detail in figure 5. Figure 6 shows a logic algorithm diagram supporting the model.
Figure 5. RQVSP model
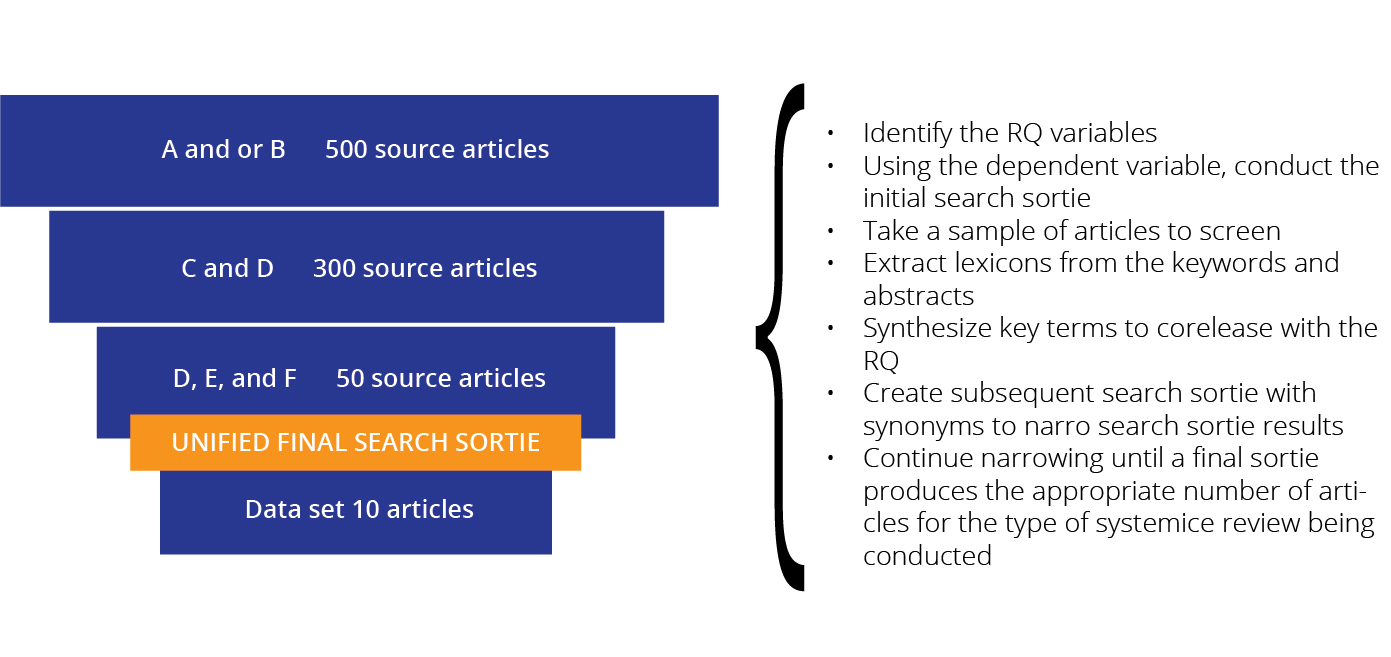
Source: courtesy of the author, adapted by MCUP.
Figure 6. Conceptual algorithm
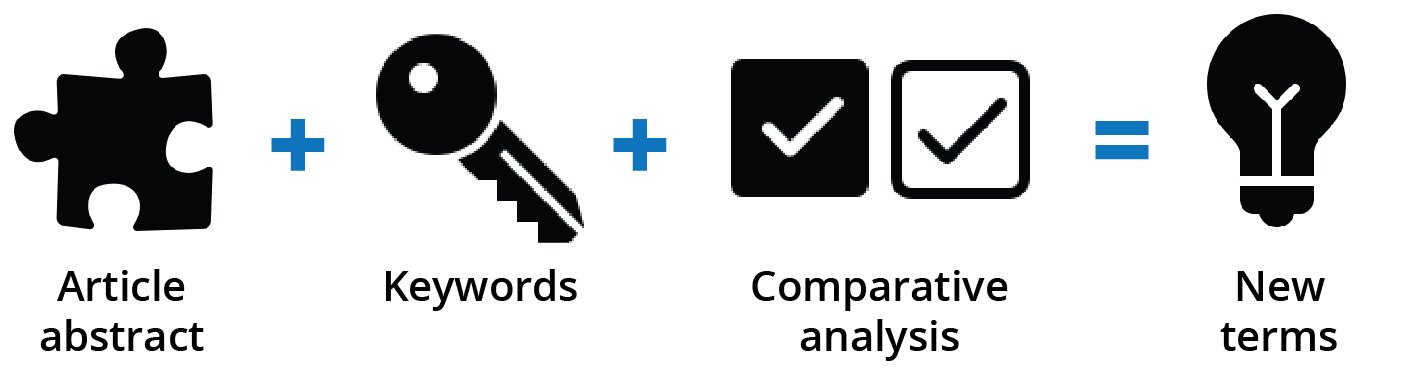
Source: courtesy of the author, adapted by MCUP.
The RQVSP model is based on semantics—specifically, the analysis of the research question’s sentence structure to identify the variables. These variables are typically written as subjects and direct objects. In a well-developed research question, the logical sequence will show A influencing B, where A is the subject of the sentence and B is the direct object. The key to identifying these variables is to look for this sequence within the research question and identify A and B, which become the variables for the initial search sortie. Synonyms for subsequent sorties are developed by analyzing the abstracts and key terms of the articles returned from the initial sortie. This process continues until the desired data set is revealed.
In most areas of research, the initial sortie/query containing the research question variables will return at least several hundred articles of scholarship. This necessitates the requirement for narrowing the number of articles identified in each sortie. In corporate research processes for decision making, the number of required articles in a data set could be as low as three. In systematic review formats such as the CAT or rapid assessment of evidence (RAE), the average number of articles in a data set is between 7 and 10. A dissertation data set requires a minimum of 30 articles. The process of narrowing the results of the initial sortie to the number appropriate for each of the aforementioned data sets is crucial.
Although it is possible to screen the abstracts and key terms of several hundred articles, there are reasons not to do so. Synthesizing and summarize the abstracts and key terms of several hundred articles of scholarship is time consuming. In addition, doing so would create a supplemental knowledge management problem, meaning that the analysis and synthesis of hundreds of articles would increase the scope of any systematic review beyond its intended purpose.49 Each source would have to be evaluated separately in a quality appraisal. Each source requires coding and a thematic analysis and synthesis to create evidence for decision making. The scope of such an effort would be too vast for an article in a peer reviewed journal, thereby defeating the purpose of a systematic review.
For transparency’s sake, it is important to capture the constructs of each search sortie. The constructs to capture from each sortie are as follows: the order in which the search sortie was executed; the terms used in each search sortie; Boolean connectors used; and the number of articles identified by each sortie.
Recording searches in a systematic review is often done in a matrix that is accompanied by a primary reporting item for systematic review and meta-analysis (PRISMA) diagram. However, in this prototype, the essences of the matrix and PRISMA diagram are combined and presented in a standard funnel chart. The funnel chart used in this prototype captures the key constructs depicted in the example in figure 5.
The information captured in a funnel chart tells the story of the search. The chart identifies the narrowing process and how it was derived from the initial research question variables. The transparency protocols within the RQVSP can be validly replicated. By using the recorded terms and connectors, if the data bases and interfaces are the same, the search will identify the same articles. The rigor of this RQVSP ensures that bias is concentrated in the narrowing protocols where sampling occurs. The rigor, transparency, and valid replication protocols within this prototype lend reliability to any study in which it is employed.
Summary
As discussed above, this article is the third in a series of four. This article conceptualized the process of building data sets for systematic reviews as part of a larger concept of integrating the EBF with MJDM. The literature review herein revealed studies that speak to the interfaces and practices of locating data. The literature, however, was deficient in outlining specific protocols for acquiring data that would enhance the rigor, transparency, validity, and reliability of the systematic review. To that end, design thinking methodologies were applied to the deficiencies identified in the literature review. The results of this effort were threefold. First, protocols were produced by the author’s team using the Stanford Design Thinking Process model. Second, these protocols function to guide the scholar practitioner through a process specifically designed for an integration of the EBF and MJDM. Finally, the protocols contain specific protocols to enhance rigor, transparency, validity, and reliability of the systematic review. In the final article in this series, the appraisal of data sets will be discussed.
Endnotes
- See David E. McCullin, “Exploring Evidence-Based Management in Military Planning Processes as a Critically Appraised Topic,” Expeditions with MCUP, 16 July 2021, https://doi.org/10.36304/ExpwMCUP.2021.04.
- See David E. McCullin, “The Integration of the Evidence-Based Framework and Military Judgment and Decision-Making,” Expeditions with MCUP, 10 November 2021, https://doi.org/10.36304/ExpwMCUP.2021.07.
- Eric Barends, Denise M. Rousseau, and Rob B. Briner, Evidence-Based Management: The Basic Principles (Amsterdam, Netherlands: Center for Evidence-Based Management, 2014), 4.
- Framework for Information Literacy for Higher Education (Chicago, IL: Association of College and Research Libraries, 2016), 8.
- Barends, Rousseau, and Briner, Evidence-Based Management.
- Barends, Rousseau, and Briner, Evidence-Based Management, 5.
- Cynthia Grant and Azadeh Osanloo, “Understanding, Selecting, and Integrating a Theoretical Framework in Dissertation Research: Creating the Blueprint for Your ‘House’,” Administrative Issues Journal: Connecting Education, Practice, and Research 4, no. 2 (2014): 13, https://doi.org/10.5929/2014.4.2.9.
- Shaul Dar et al., “DTL’s DataSpot: Database Exploration Using Plain Language,” in VLDB ’98: Proceedings of the [24th] International Conference on Very Large Data Bases, August 24–27, 1998, New York City, New York, USA, ed. Ashish Gupta, Oded Shmueli, and Jennifer Wisdom (San Francisco, CA: Morgan Kaufmann Publishers, 1998), 648.
- Margaret J. Foster and Sarah T. Jewell, eds., Assembling the Pieces of a Systematic Review: A Guide for Librarians (Lanham, MD: Rowman & Littlefield, 2017), 38.
- Grant and Osanloo, “Understanding, Selecting, and Integrating a Theoretical Framework in Dissertation Research.”
- Harold E. Briggs and Bowen McBeath, “Evidence-Based Management: Origins, Challenges, and Implications for Social Service Administration,” Administration in Social Work 33, no. 3 (2009): 245–48, https://doi.org/10.1080/03643100902987556.
- “Systematic Reviews and Other Review Types,” Temple University Libraries, accessed 14 October 2021.
- Joint Targeting, Joint Publication (JP) 3-60 (Washington, DC: Joint Chiefs of Staff, 2013), 1-30.
- Daniel Hienert, Frank Sawitzki, and Philipp Mayr, “Digital Library Research in Action: Supporting Information Retrieval in Sowiport,” D-Lib Magazine 21, no. 3/4 (March/April 2015), https://doi.org/10.1045/march2015-hienert.
- Dion Hoe-Lian Goh et al., “A Checklist for Evaluating Open Source Digital Library Software,” Online Information Review 30, no. 4 (July 2006): 360–79, https://doi.org/10.1108/14684520610686283.
- Muhammad Rafi, Zheng Jian Ming, and Khurshid Ahmad, “Evaluating the Impact of Digital Library Database Resources on the Productivity of Academic Research,” Information Discovery and Delivery 47, no. 1 (December 2018): 42–52, https://doi.org/10.1108/IDD-07-2018-0025.
- Krutarth Patel et al., “Keyphrase Extraction in Scholarly Digital Library Search Engines,” in ICWS Web Services: ICWS 2020, ed. Wei-Shinn Ku et al., Lecture Notes in Computer Science Series, vol. 12406 (Berlin: Springer, 2020), 179–96, https://doi.org/10.1007/978-3-030-59618-7_12.
- Paula Younger and Kate Boddy, “When Is a Search Not a Search?: A Comparison of Searching the AMED Complementary Health Database via EBSCOhost, OVID and DIALOG,” Health Information and Libraries Journal 26, no. 2 (June 2009): 126–35, https://doi.org/10.1111/j.1471-1842.2008.00785.x.
- Dar et al., “DTL’s DataSpot.”
- Maureen O. Meade and W. Scott Richardson, “Selecting and Appraising Studies for a Systematic Review,” Annals of Internal Medicine 127, no. 7 (October 1997): 531–37, https://doi.org/10.7326/0003-4819-127-7-199710010-00005.
- Sherry E. Mead et al., “Influences of General Computer Experience and Age on Library Database Search Performance,” Behaviour and Information Technology 19, no. 2 (March 2000): 107–23, https://doi.org/10.1080/014492900118713.
- Rosalind F. Dudden and Shandra L. Protzko, “The Systematic Review Team: Contributions of the Health Sciences Librarian,” Medical Reference Services Quarterly 30, no. 3 (July 2011): 301–15, https://doi.org/10.1080/02763869.2011.590425.
- Susan A. Murphy and Catherine Boden, “Benchmarking Participation of Canadian University Health Sciences Librarians in Systematic Reviews,” Journal of the Medical Library Association 103, no. 2 (April 2015): 73–78, https://doi.org/10.3163/1536-5050.103.2.003.
- Aldemar Araujo Castro, Otávio Augusto Câmara Clark, and Álvaro Nagib Atallah, “Optimal Search Strategy for Clinical Trials in the Latin American and Caribbean Health Science Literature Database (LILACS Database): Update,” Sai Paulo Medical Journal 117, no. 3 (May 1999): 138–39, https://doi.org/10.1590/S1516-31801999000300011.
- Wichor M. Bramer et al., “De-Duplication of Database Search Results for Systematic Review in EndNote,” Journal of the Medical Library Association 104, no. 3 (July 2016): 240–43, https://doi.org/10.3163/1536-5050.104.3.014.
- Jan Brophy and David Bawden, “Is Google Enough?: Comparison of an Internet Search Engine with Academic Library Resources,” Aslib Proceedings 57, no. 6 (December 2005): 498–12, https://doi.org/10.1108/00012530510634235.
- Lov K. Grover, “A Fast Quantum Mechanical Algorithm for Database Search,” in STOC ’96: Proceedings of the Twenty-Eighth Annual ACM Symposium on Theory of Computing, ed. Gary L. Miller (New York: Association for Computing Machinery, 1996), 212–19, https://doi.org/10.1145/237814.237866.
- Jody Condit Fagan, “Usability Testing of a Large, Multidisciplinary Library Database: Basic Search and Visual Search,” Information Technology and Libraries 25, no. 3 (September 2006): 140–50, https://doi.org/10.6017/ital.v25i3.3345.
- Bramer et al., “De-Duplication of Database Search Results for Systematic Reviews in Endnote,” 240–43.
- Hienert, Sawitzki, and Mayr, “Digital Library Research in Action.”
- Barends, Rousseau, and Briner, Evidence-Based Management, 12–14.
- Edoardo Aromataris and Dagmara Riitano, “Constructing a Search Strategy and Searching for Evidence: A Guide to the Literature Search for a Systematic Review,” American Journal of Nursing 114, no. 5 (May 2014): 49–56, https://doi.org/10.1097/01.NAJ.0000446779.99522.f6.
- Nikhil Mahen, “Systems Thinking vs. Design Thinking: A Spectrum Misunderstood,” Medium, 5 October 2020.
- David Terrar, “What Is Design Thinking?,” Agile Elephant (blog), 18 February 2018.
- Mahen, “Systems Thinking vs. Design Thinking.”
- Mahen, “Systems Thinking vs. Design Thinking.”
- Phanish Puranam et al., “Modelling Bounded Rationality in Organizations: Progress and Prospects,” Academy of Management Annals 9, no. 1 (2015): 337–92, https://doi.org/10.5465/19416520.2015.1024498.
- Mahen, “Systems Thinking vs. Design Thinking.”
- Mahen, “Systems Thinking vs. Design Thinking.”
- Mahen, “Systems Thinking vs. Design Thinking.”
- See McCullin, “Exploring Evidence-Based Management in Military Planning Processes as a Critically Appraised Topic.”
- See McCullin, “The Integration of the Evidence-Based Framework and Military Judgment and Decision-Making.”
- See McCullin, “The Integration of the Evidence-Based Framework and Military Judgment and Decision-Making.”
- Joint Targeting, B-2.
- Joint Targeting, 1-33.
- Joint Targeting, 1-34.
- Joint Targeting, 1-36.
- See McCullin, “Exploring Evidence-Based Management in Military Planning Processes as a Critically Appraised Topic”; and McCullin, “The Integration of the Evidence-Based Framework and Military Judgment and Decision-Making.”
- The standard sample size for a normalized data set is usually at least 30. In practice, the number is closer to 10, as in a critically appraised topic (CAT) or rapid assessment of evidence (RAE). Cost and time usually prevent the use of more that 10 studies.