David E. McCullin, DM
28 September 2023
https://doi.org/10.36304/ExpwMCUP.2023.12
PRINTER FRIENDLY PDF
EPUB
AUDIOBOOK
Abstract: This article is the final entry in a four-part series that discusses the integration of the evidence-based management (EBM) framework with the military judgement and decision making (MJDM) process as explained in Joint Planning, Joint Publication (JP) 5-0. The instructional nature of this article is intentional. The instructions provided herein are meant to supplement the first article in the series, which demonstrated that an integration of EBM and MJDM is feasible. These innovations build on the first article with practical methods of mitigating bias in the appraisal process. This article makes three propositions: first, that the limits of human cognition manifest as bias in decision-making processes; second, that there is an indirect relationship between time and efficiency lost by the limits of human cognition; and third, that within the EBM-MJDM integration, bias can be mitigated in the appraisal process that is integrated in the systematic review. Using these propositions, this article appraises the quality of scholarship, organizational data, subject matter expertise, and stakeholder input from the EBM framework into the Joint planning processes outlined in JP 5-0. The propositions were the basis of specific recommendations on appraising data specific to the EBM-MJDM integration.
Keywords: informational appraisals, evidence-based management, military judgement and decision-making, military planning, Joint planning process, selection bias, bounded rationality
This article is the final entry in a four-part series that discusses the integration of the evidence-based management (EBM) framework with the military judgement and decision making (MJDM) process. The first article in this series presented research on the feasibility of an EBM-MJDM integration. That study used a critically appraised topic (CAT)—a systematic review methodology—to explore the potential of integration. The findings of the CAT demonstrated that the integration was both feasible and practical. As a result of that study, three additional articles were planned to conceptualize how such an integration might be implemented.1 The second article focused on how this integration could occur at the Joint planning level.2 The third article explained the integration in terms of applying information literacy to build data sets.3 This series also offers a response to U.S. president Joseph R. Biden Jr.’s 2021 directive on restoring trust in government through scientific integrity and evidence-based policymaking, which outlined new organizational decision-making policies and procedures that all government agencies are mandated to follow.4
Definitions
The following definitions are provided here to offer context and clarity for the terms used in this article. They represent a compilation of well-accepted theories and practices.
1. Critically appraised topic (CAT): provides a quick and succinct assessment of what is known (and not known) in the scientific literature about an intervention or practical issue by using a systematic methodology to search and critically appraise primary studies.5
2. Data set: information collected from stakeholders, subject matter experts, organizational data, and scholarship. The aggregation of each source is a separate data set.
3. Research question: a question developed from a pending decision to focus a research effort that is designed to create evidence.6
4. Evidence-based management (EBM): a decision-making framework that draws evidence from experience, stakeholder input, organizational data, and scholarship.7
5. Evidence-based practice (EBP): the employment of a methodology of asking, acquiring, appraising, aggregating, applying, and assessing.8
6. Stakeholders: individuals or organizations directly impacted by a judgment or decision.9
7. Military judgment and decision making (MJDM): a spectrum of decision-making processes related to the arts and sciences of national defense. Within this spectrum, quantitative and qualitative processes are used to make decisions based on multiple courses of action.
8. Theoretical framework: links theory and practice. The author of a study selects one or more theories of social science research to help explain how a study is linked to a practical approach identified in a research question.10
9. Bounded rationality: the limited capacity of human cognition in acquiring and processing information.11
10. Conceptual framework: an explanation of how the constructs of a study are strategically used in addressing the research question. A theoretical framework is often accompanied by a sketch showing how the constructs of a study are related.12
11. Organizational complex adaptive systems (OCAS): are made up of elements such a material, labor, and equipment and are guided by specified actions. OCAS occur when organizations create habitual networks of elements to accomplish a societal goal. They face societal pressures to constantly evolve. They are vulnerable to constraints that impede systems functioning, which causes the elements to adapt the system.13
12. Data points: short text passages, phrased in as few words as possible, that guide the task of collecting and categorizing nonstandard data.
Introduction
There are enough examples of bad decisions that were made based on biased evidence to make a strong argument that the consequences of such decisions can range from embarrassing to disastrous. The dangers of biased information play out in many arenas—from national elections to court decisions, from public policy to military planning, and in economic life from mergers and acquisitions to individual purchases. One example in economic life is that of the web portal Excite, which passed up a chance to buy Google for $750,000 in 1999. The Daimler-Benz merger, the Kodak digital camera failure, the Blockbuster video decline, junk bonds, Ponzi schemes, and multilevel marketing companies such as Amway all demonstrate examples of the dangers of relying on biased information. In the realm of policy making, there was the rollout of the polio vaccine, the Great Depression, and Jim Crow laws. Militarily, there was Pickett’s Charge at the Battle for Gettysburg, the failed Bay of Pigs operation, and numerous decisions made during the Vietnam War. All of these examples demonstrate scenarios in which dangers of biased information negatively impacted lives, often with dire consequences.
The EBM framework and MJDM process were both designed to create evidence for decision making in which rigor transparency, validity, and reliability mitigate bias in information. In an EBM-MJDM integration, the systematic review is the conduit for the integration. The systematic review reports on asking, acquiring, appraising, aggregating, and applying evidence for decision making. That is, asking a specific question that guides the research effort; acquiring the information to answer the research question; appraising the quality of the information and its source; aggregating information to create evidence; and applying the evidence to support decision making. This article is concerned with the “appraising” stage of the systematic review and introduces methods of mitigating bias by leveraging the appraisal process.
Figure 1. EBM-MJDM integration model
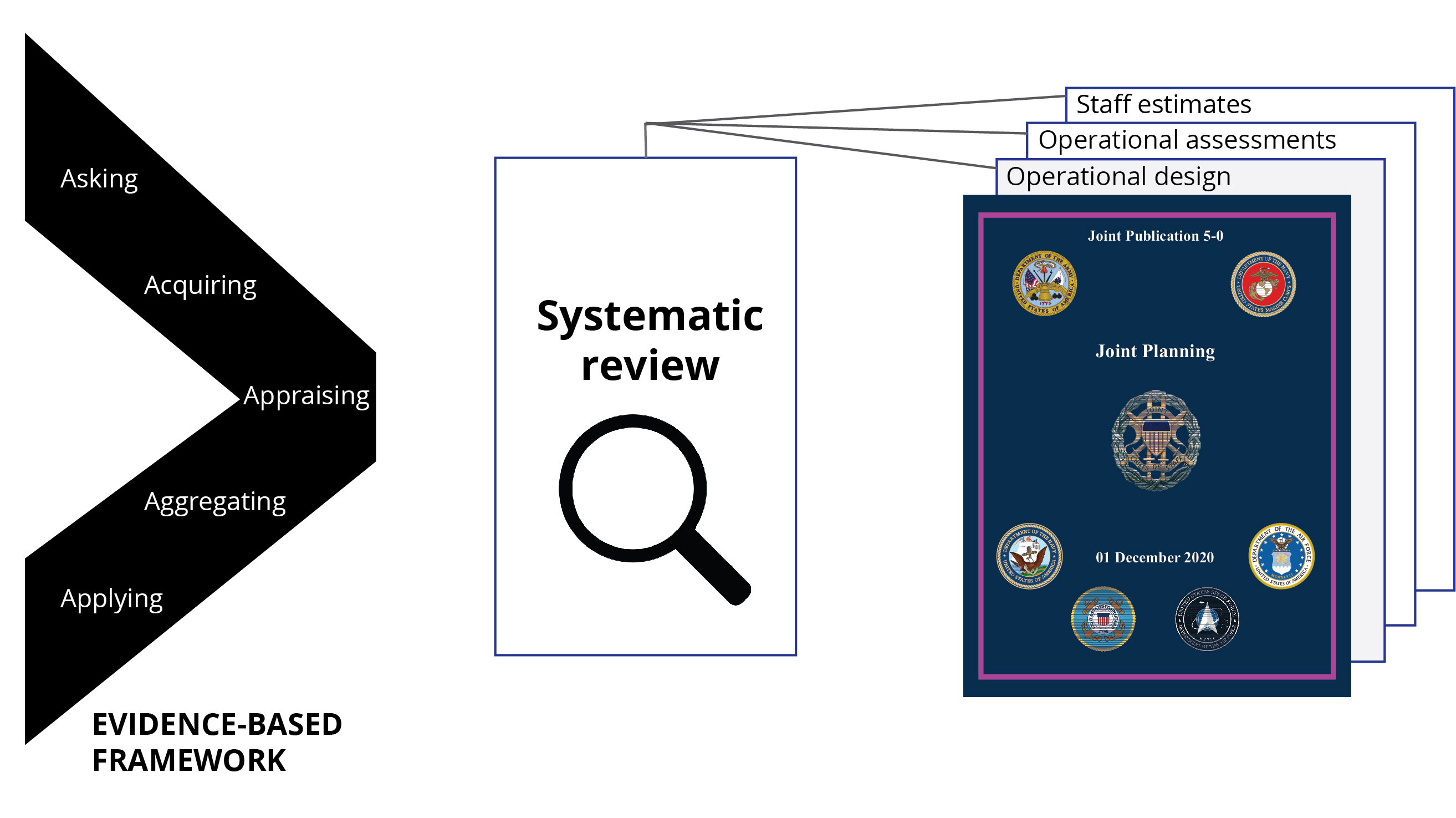
Source: courtesy of the author, adapted by MCUP.
Figure 1 depicts the EBM-MJDM integration as described in the second article in this series.14 As shown, the integration occurs by using systematic reviews to supplement operational design, assessments, and estimates in the Joint planning processes explained in Joint Planning, Joint Publication (JP) 5-0.15 The critically appraised topic, the rapid assessment of evidence, the systematic review of literature, and the meta-analysis all incorporate requirements for asking, acquiring, appraising, aggregating, and applying. Therefore, any of these formats can be used to leverage the appraisal process to mitigate bias through the use of rigor, transparency, validity, and reliability as appraisal criteria. Figure 2 depicts the leveraging of the appraisal process required in systematic reviews to mitigate bias using validity, reliability, rigor, and transparency to appraise human and institutional data as explained in this article.
Figure 2. Leveraging appraisals to mitigate bias
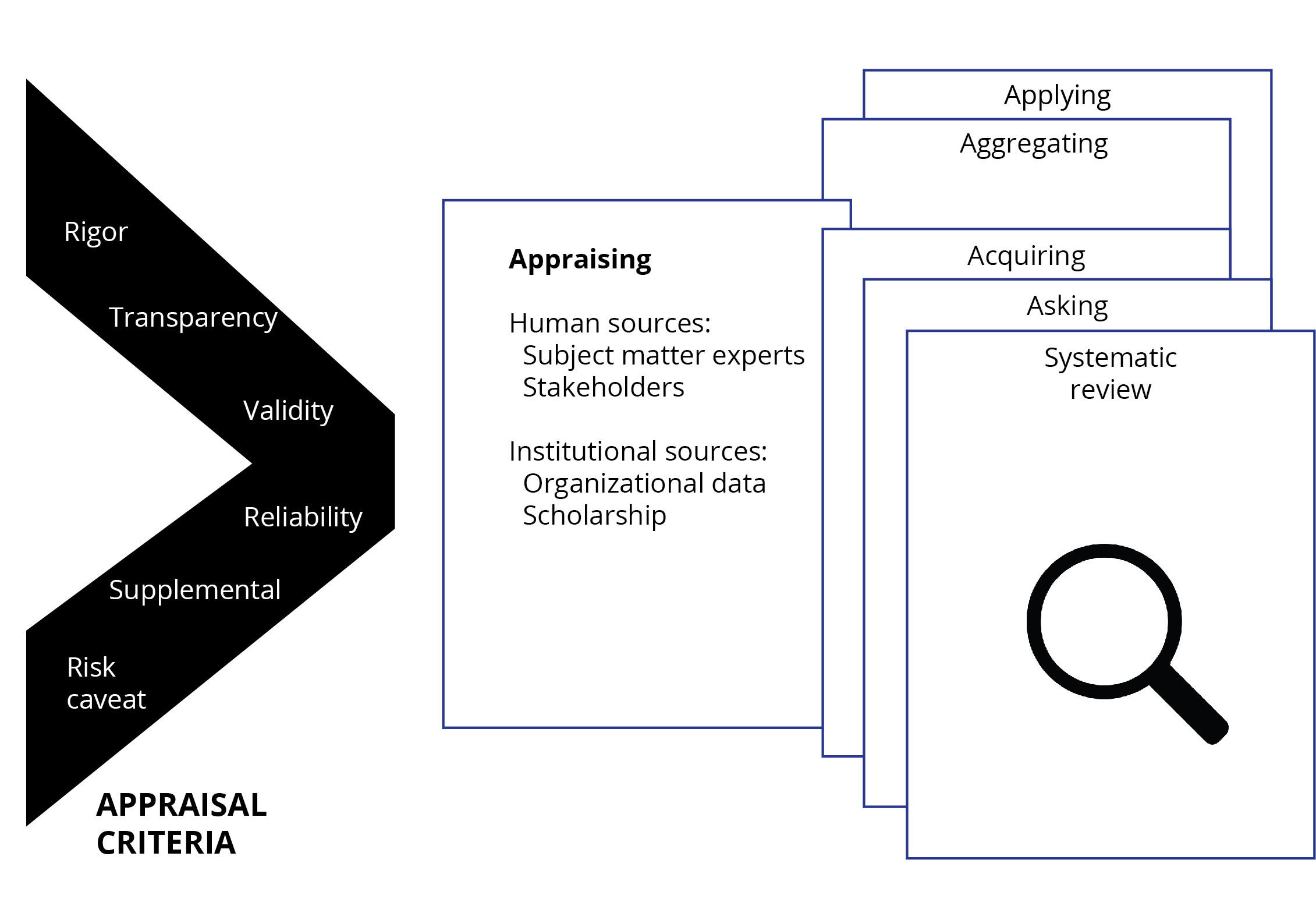
Source: courtesy of the author, adapted by MCUP.
The purpose of this article is three-fold: first, to provide background on the importance of information taxonomy in appraising information within the evidence-based framework (EBF); second, to discuss how the “appraising” section of the systematic review is leveraged to mitigate bias; and third, to introduce and explain specific procedures that can be used to leverage appraisals to mitigate bias.
Information Taxonomy and Appraisal within the Evidence-Based Framework
Before making an important decision, an evidence-based practitioner begins by asking, “What is the available evidence?”16 Integrating the EBM framework into MJDM methodologies is a complex adaptive system executed as an operational art. The EBF seeks evidence from subject matter experts, stakeholder input, organizational data, and scholarship. The information taxonomy as described in this series of articles further classifies EBF sources as human and institutional. The articles also recognize that scholarship is the single informational component within the EBM framework that is not currently integrated into military planning as an operational art.
Context of the Four-Article Series
Instead of basing a decision on personal judgment alone, an evidence-based practitioner finds out what is known by looking for evidence from multiple sources.17 The recommendations in the first article in this series suggested the emergence of scholar practitioners within the U.S. Department of Defense to execute the operational arts associated with the EBM-MJDM integration. The second article specifically established the systematic review as the mechanism for implementing the EBF in an EMB-MJDM integration and named the military planning scholar practitioner as the implementor. Together the first two articles also established that the best available evidence is created by the judicious use of information accomplished through the EBF, which creates evidence by asking, acquiring, appraising, aggregating, applying, and assessing information.18
All evidence should be critically appraised by carefully and systematically assessing its trustworthiness and relevance.19 This statement on the trustworthiness of evidence epitomizes the importance of the EBM framework in decision-making processes. The strength of the EBM-MJDM integration proposed herein rests on the trustworthiness of evidence. Trustworthiness in evidence is established through the rigor and transparency of the appraisal process, meaning that a strong appraisal will give the decision maker the full and transparent spectrum of how the evidence was appraised.
In the systematic review’s appraisal process, the data set is transparently displayed with all relevant information categorized. The appraisal focuses on the quality of the data collected and its relevance to answering a predetermined research question. There are numerous methods that can be used to assess the quality and relevance of a data set. The key is to assess each source in a process that demonstrates rigor and transparency. During the appraisal, judgements are made that may eliminate studies from the data set because of low quality or a weak nexus to the research question.
Taxonomy and Information Appraisal
As previously mentioned, within the EBF and the systematic review, evidence is created from four categories, and within this series the taxonomy of information further categorizes these sources as institutional and human. Institutional categories include organizational data and scholarship, such as science from original research. Human categories include subject matter expertise and stakeholder input. The informational sources and categories are both supplementary and complementary. These factors are shown in figure 3.
Figure 3. Information taxonomy
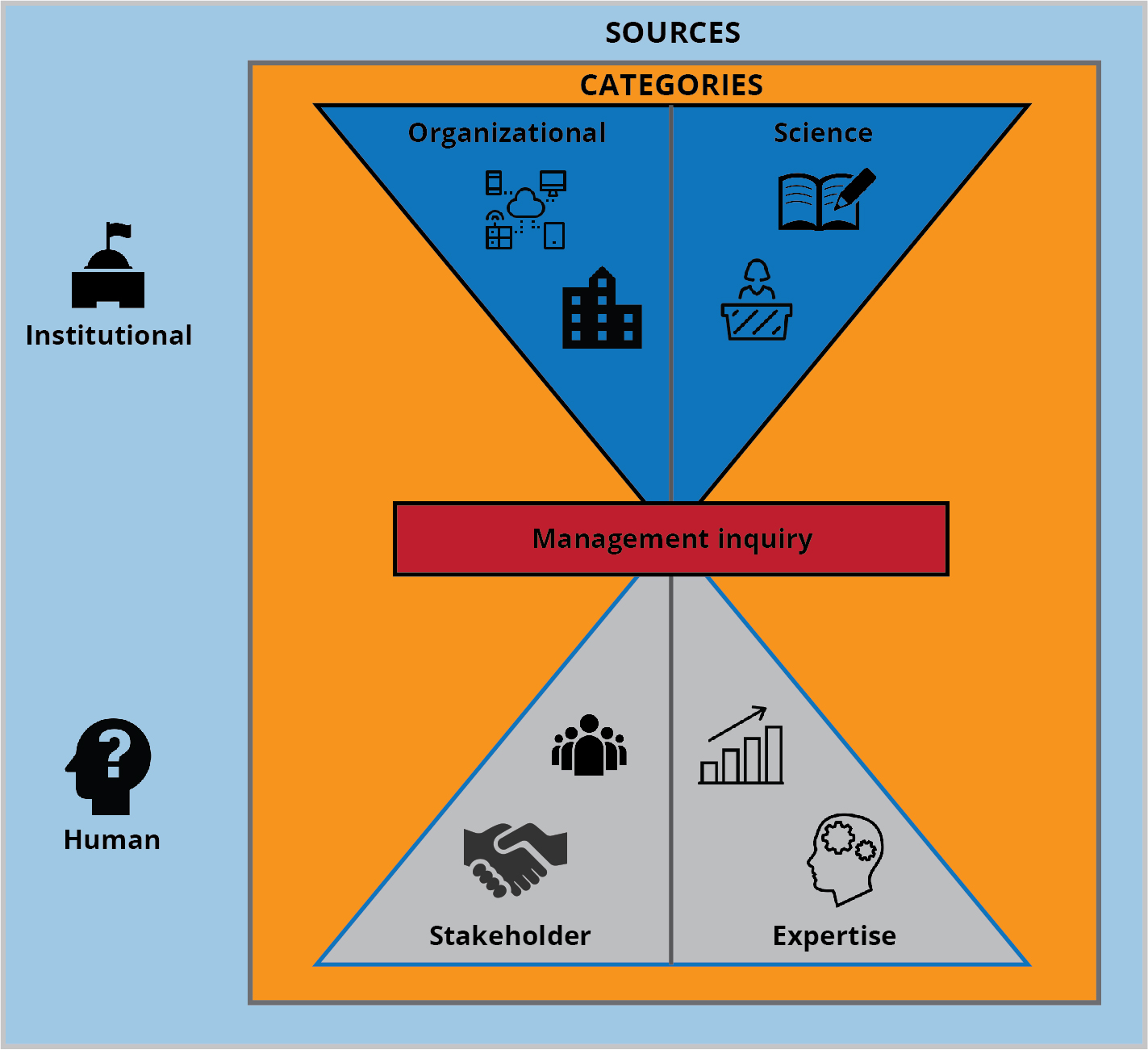
Source: courtesy of the author, adapted by MCUP.
The taxonomy supplements the types of information needed by suggesting where information may be located, how to obtain it, what the appraisal strategy will be, and where bias is to be expected. The unmistakable distinction between human and institutional information is their primary and secondary characteristics. Secondary characteristics apply to organizational data and scholarship, which is information that already exists and can be obtained from an archive. As human source information is collected from subject matter experts and stakeholders, it is collected as a primary source before it is archived. Based on these characteristics, the collection and appraisal strategies for these two sources will differ.
The informational categories are complementary in that any single source of information can validate or invalidate any other source. This design provides a decision-making panacea. In order to locate and collect the information needed, the human and institutional sources must be carefully considered. In order to fully implement the EBM framework information, all four categories must be represented in the final decision. According to Eric Barends, Denise M. Rousseau, and Rob B. Briner, a critical evaluation of the best-available evidence, as well as the perspectives of those people who might be affected by the decision, epitomizes the core concept of EBM.20
In this series, the information taxonomy guides the search process and evaluation methodology. It also indirectly suggests the type of instrument needed to collect data. The collection instruments used here are surveys and checklists to collect data from human sources and search strings and templates for analysis and synthesis to collect archived data. By using the taxonomy as a guide, the practitioner can ensure that all information from human and institutional categories are represented in the final data set.
Propositions
This article makes three propositions based on the axiom that decision making is a form of human cognition. The first is that the limits of human cognition manifest as bias in decision-making processes. The second is that there is an indirect relationship between time and efficiency lost by the limits of human cognition. The third is that within the EMB-MJDM integration, bias can be mitigated in the appraisal process that is integrated in the systematic review. In this article, the theoretical frameworks for mitigating human source bias are bounded rationality and the availability heuristic.21
The limits of human cognition herein are explained as bounded rationality, which assumes that “the decision-making process begins with the search for alternatives”; that “the decision maker has egregiously incomplete and inaccurate knowledge about the consequences of actions”; and that “actions are chosen that are expected to be satisficing (attain[ing] targets while satisfying constraints).”22
This article introduces a unique conceptual approach to appraising human sources. The theoretical framework for this concept is based on the availability heuristic, which speaks to the limits of human cognition.23 A heuristic is a mental shortcut that allows an individual to make a decision, pass judgment, or solve a problem quickly and with minimal mental effort. While heuristics can reduce the burden of decision making and free up limited cognitive resources, they can also be costly when they lead individuals to miss critical information or act on unjust biases.24 The propositions behind the conceptual approach to appraising human source data introduced here is that bias is an inherent part of the human condition. Accepting this allows bias to be transparently addressed in any appraisal algorithm.
The assumption of bias places no blame for duplicitous intent on the stakeholder, subject matter expert, or any other practitioner. One of the propositions presented here is that there is an indirect relationship between time and any efficiency lost by the limits of human cognition—that is, that the more time is allotted to decision making, the less human cognition matters. In that view, bias is mitigated when there is time to apply decision-making methodologies that rely heavily on the use of acquisition and analysis of information. However, because time constraints are commonplace, the informational inputs for decision making become limited. Therefore, the assumption here is that bias in decision making is unavoidable. According to Amos Tversky and Daniel Kahneman, “Most important decisions are based on beliefs concerning the likelihood of uncertain events such as the outcome of an election, the guilt of a defendant, or the future value of the dollar. These beliefs are usually expressed in statements such as ‘I think that . . .’, ‘chances are…’, [and] ‘It is unlikely that . . .’.”25 The uncertainty expressed in this quote originates from a void of evidence. For this reason, decision-making practitioners and processes such as MJDM and EBM were developed.
This quote suggests the complexity of organizational decision making in the face of uncertainty. As indicated therein, evidence is created with expressions of belief that are given to bounded rationality. The EBM framework and MJDM process are both organizational complex adaptive systems that account for uncertainty in decision making in planning purposes that are not given to bounded rationality. This article hypothetically links uncertainty to the escalation of bias.26
Making decisions with the best available evidence is a key component in both the EBM framework and MJDM process. The appraisal of evidence to determine its relevance to a question is a component of EBM brought to MJDM through the integration. In addition to appraising evidence for quality, this article uses the propositions of bias to identify where bias may occur in the evidence. To that end, the article introduces methods of appraisal to mitigate bias in the evidence. The methods espoused herein add rigor to decision making processes while also mitigating bias through the appraisal process.
Appraisal Criteria
In this article, the process of developing criteria incorporates three considerations: versatility, objectivity, and unambiguousness. Versatile criteria facilitate the appraisal process by providing a metric that logically measures the quality of both human and institutional sources equally. Objective criteria provide a metric that reduces biases in the evaluation process. Unambiguous criteria are clearly and universally interpreted based on established conceptualizations. These considerations are realized as methodologically appropriate. The practitioner must seek methodologically appropriateness as criteria are being developed.
There are a myriad of theories and concepts to guide the practitioner in ensuring that methodological appropriateness is inherent in the appraisal criteria. Within the EBM framework, there are four sources of data that can be added to a systematic review: subject matter experts, stakeholders, organizational data, and scholarship. The taxonomy herein further categorizes the data as human and institutional sources.
As mentioned, the information taxonomy can be used to help the practitioner visualize the types of bias that originate from the human element that is present in all data sources. A full discussion of the terms of appraisal methodology occurs in the following section of this article. However, to preface that discussion, it is critical to understand that the antecedent of appraising information is to choose or develop a set of appraising criteria. The information taxonomy guides this effort. Because information in this series is categorized as human or institutional, the recommended strategy is to use criteria lucid enough to appraise both informational categories: rigor, transparency, validity, and reliability. These criteria do not represent an all-inclusive list but can be used as a basic assessment for all categories associated with the EBF. They can also be appended with additional criteria. These criteria are well-established, well-understood, and well-practiced as evaluating criteria in academic research. Although they are extensively used to appraise scholarship, they are recommended herein because of their lucidity and flexibility. They are recommended at minimum but may be expanded on as appropriate at the discretion of the practitioner. This article expands on the basic criteria by introducing a risk caveat, which is explained in detail below.
Rating Criteria
Although the criteria detailed above provide the means to measure quality of data sets, rating scales provide the metric for each criterion. Rating scales are used to measure the levels of each criteria within each observation of data. Two commonly used scales are the linear numeric scale and the Likert scale.
Figure 4. Linear numeric and Likert scales
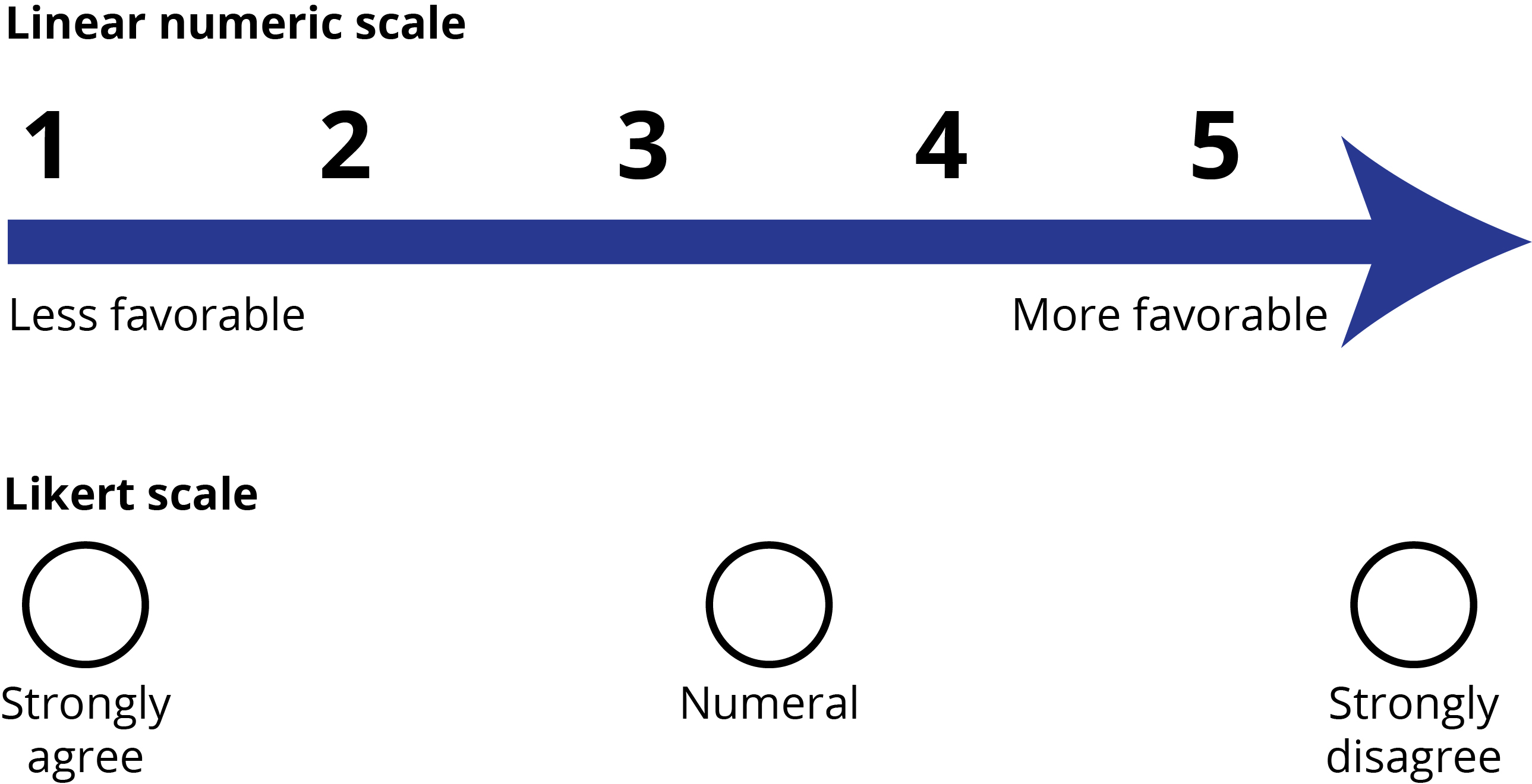
Source: courtesy of the author, adapted by MCUP.
Figure 4 depicts examples of a linear numeric scale and a Likert scale. These scales are both universally accepted and widely used. They can be used to appraise rigor, transparency, validity, and reliability in both human source data and institutional data. It is incumbent upon the practitioner to consider the appropriate rating scale when designing surveys, observations, and/or search strategies.
The Risk Caveat
The risk caveat was specifically designed for the EBM-MJDM integration. It assesses the risk of accepting bias in a data set. By default, the risk caveat also assesses risk associated with not following the recommendations supported by evidence. Applying the risk caveat can be range for a mental process to a collaboration. The complete application of the risk caveat is given in the logic model in figure 5.
Figure 5. Risk caveat logic model
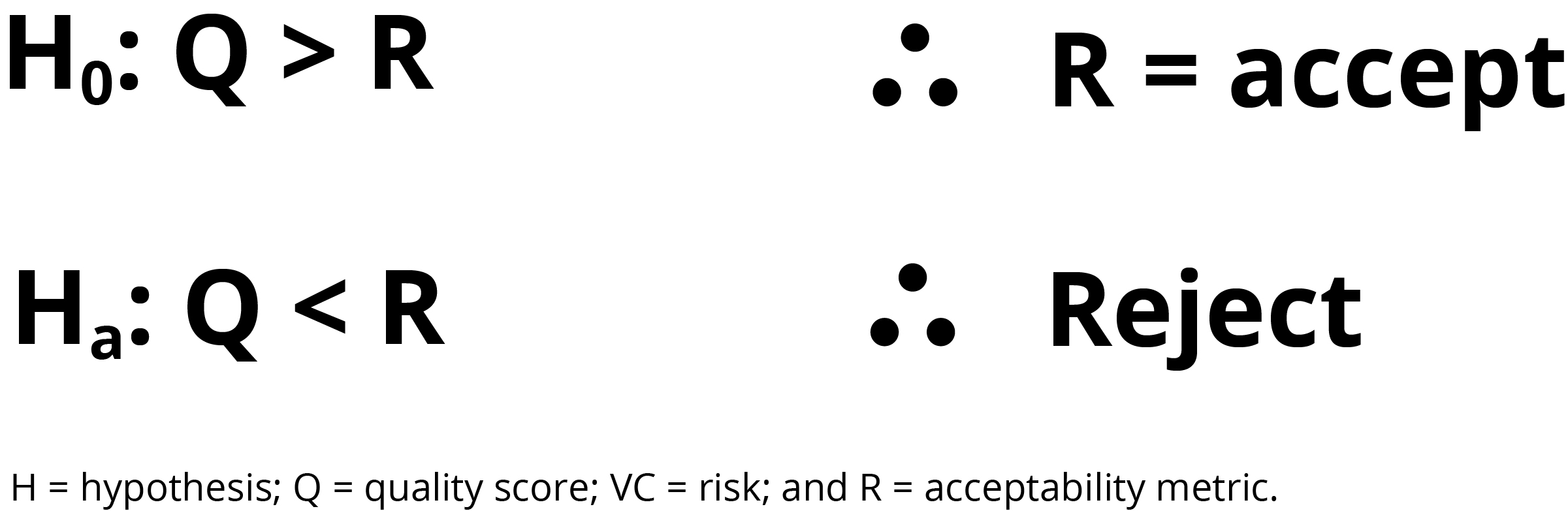
Source: courtesy of the author, adapted by MCUP.
In this model, the null hypothesis is that the assessed level of risk in the data is acceptable. The acceptable level of risk is determined by “Q"—the overall level of rigor, transparency, validity, and reliability in the data. The higher the Q level, the lower the risk. To apply the risk caveat, the practitioner uses the following steps:
1. Appraise each data source for rigor, transparency, validity, and reliability.
2. Determine the Q score.
3. Determine the acceptability metric.
4. Judge the acceptability of the hypotheses.
The acceptability metric is an expression of risk that is identified on a linear numeric scale or a Likert scale and categorized. For example, assessment of risk can be categorized as high, medium, or low or combined with a numeric scale in which a range of numbers represent high, medium, and low levels of risk of accepting biased data. The purpose of the acceptability metric is to provide a benchmark for accepting or rejecting the null hypothesis. Accepting the null hypothesis offers an indication of acceptable level risk in the data due to bias. The decision maker ultimately decides if the acceptability metric will be higher or lower. The final determination of acceptance or rejection is made by comparing the Q score to the acceptability metric. If the Q score exceeds the acceptable level of quality identified by the acceptability metric, the null hypothesis is accepted.
In the risk caveat logic model, the Q score is determined by appraising all human and institutional data sources in which each source is scored in terms of validity, reliability, rigor, and transparency. The rating scale gives the Q its numeric value. When all the data sources have been scored, the practitioner uses the separate scores to determine an overall score of quality designated by Q. This can be done, for example, by averaging the separate scores. With rigor, transparency, validity, reliability, and the risk caveat as criteria, the appraisal process proposed herein can mitigate bias and assess quality in both human and institutional data.
Appraising the Quality of Evidence in Systematic Reviews
As explained by Guy Paré et al., the systematic review is an essential component of academic research.27 According to David Gough, the systematic review summarizes, critically reviews, analyzes, and synthesizes a group of related evidence to identify gaps and create new knowledge.28 The systematic review does this by asking, acquiring, appraising, aggregating, and applying. Each of these components represents a section within the systematic review. Each section requires discussion of the associated component. This article was primarily designed to populate the appraisal section. It incorporates validity, reliability, rigor, and transparency into the required section on appraising data in the systematic review format as evaluating criteria.
Data Collection Planning
Prior to any data appraisal, a data collection plan must be developed to facilitate the effort of acquiring data. In the following sections, the appraisal of data is discussed in detail. The taxonomy herein was designed to suggest divisions of labor associated with identifying and collecting data from human and institutional sources. Data is collected from human sources such as subject matter experts and stakeholders with observations, checklists, and surveys. Data is collected from institutional sources such as scholarship and organizations by templates using processes of analysis and synthesis. In both cases, it is critical to maintain focus on the research question. Identifying data points from the research question ensures that the data collected can address the question and any hypothetical propositions that have emerged from the question. Figure 6 depicts an example of how data points can be developed by focusing on research question variables.
Figure 6. Identifying data points
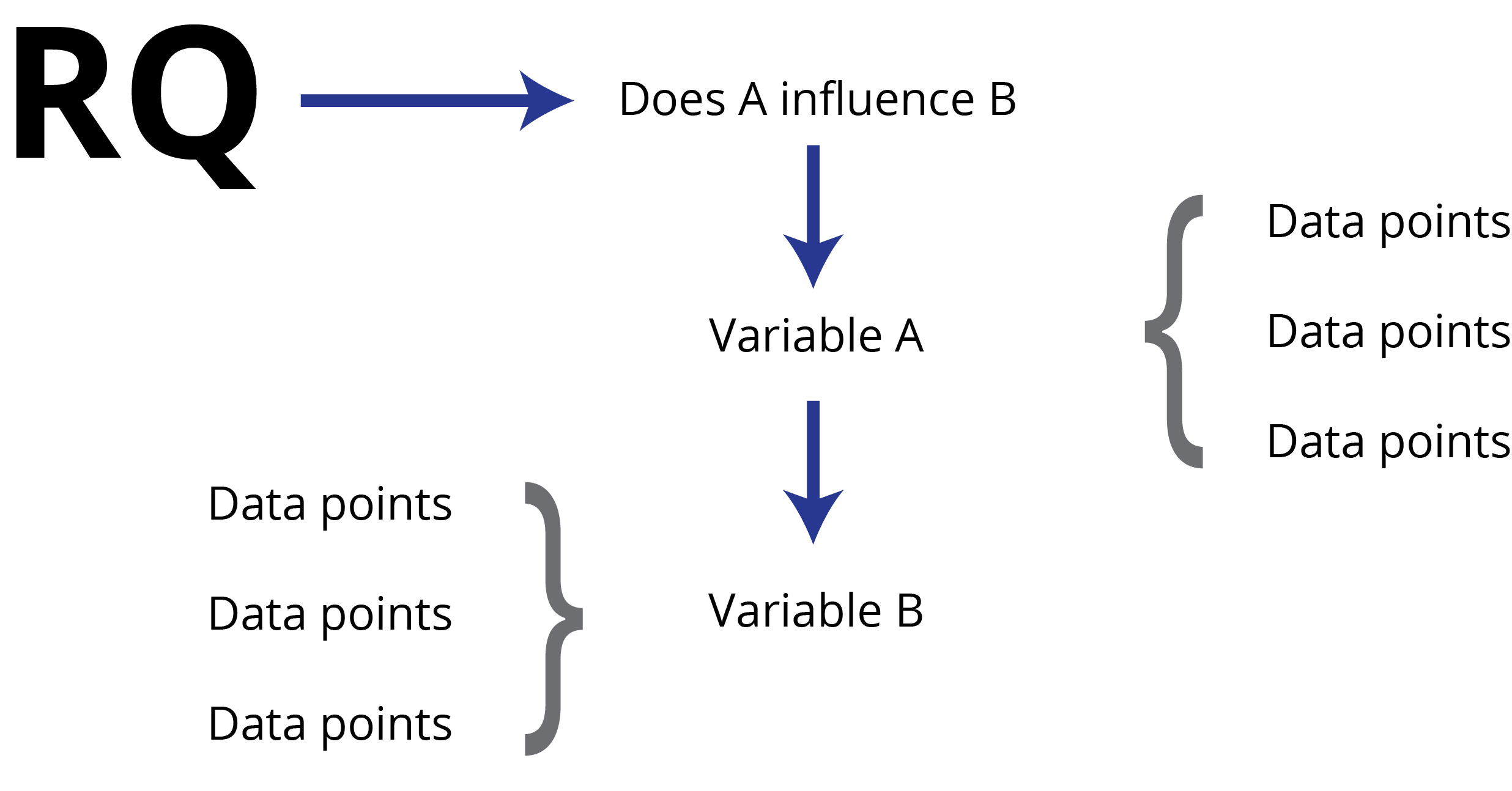
Source: courtesy of the author, adapted by MCUP.
Data points are used to collect the data that populates interface instruments such as surveys, observations, search strings, checklists, and templates used for methods of analysis and synthesis. Designing interface instruments is preceded by the development of an overall plan to collect data. A data collection plan specifically identifies the logistics of data collection. This includes permissions, scheduling, assignments, and other specific tasks associated with collecting data.
Appraising Scholarship
Scholarship is a data set of articles, usually found in peer-reviewed journals, in which each article presents a separate empirical study. Scholarship can be incorporated into the systematic review as an independent data source. Validity within an empirical study can be measured by the integrity between a clearly articulated set of findings and a research question. In the systematic review, validity can be used as a criterion to appraise the integrity between the research question and the findings. Hypothetical inquiries and/or propositions may also supplement the research question, and the integrity between them and the findings is also a consideration for appraisal in terms of validity.
Although the integrity between the research question and the findings can be measured and appraised by validity, that same integrity is explained in the methodology used in a study. How clearly and concisely the methodology is explained speaks to its rigor. What rigor specifically explains is how closely the methodology adheres to established scientific principles, which include random sampling, analysis and synthesis, and statistical tests.
In terms of transparency, a clear and transparent study will reveal the steps followed that address the research question and any supplemental hypothetical inquiries. A transparent study provides a model for replication. Appraising transparency assesses the ability of an unassociated practitioner to replicate the study using only that study as a guide. A reliable study will be generalizable. Theoretically, a reliable study will be relevant to any similar research question. For example, a study that has addressed the productivity of teleworking employees will be applicable to other similar questions on the productivity of teleworking employees. Appraising reliability involves assessing the problem area, how the research question is framed, and the rigor and validity within the study.
In the systematic review, the number of studies can range from 3 to 10. Each study must be thoroughly understood by all practitioners that provide an appraisal, and each study is to be appraised independently.
Appraising Organizational Data
Rigor, transparency, validity, and reliability can be useful as basic criteria for appraising human and institutional data. Although these criteria are lucid, they can be supplemented or appended to meet specific requirements. The systematic review provides guidance for synthesizing both human and institutional data sources into a comprehensive study. Within that guidance, the systematic review also has a requirement to appraise both institutional and human data sources. Appraising organizational data requires special consideration in terms of volume and type. The data available within an organization is vast and varied, and it must be screened to determine which records will become part of the final data set. Although screening methods were detailed in the third article in this series on information literacy, a brief summary of a screening process is provided here:29
1. Assess the complete spectrum of organizational records in terms of their potential to inform the research question.
2. Determine which records will be used.
3. Using the research question, develop the data points to be extracted from each record.
4. Format the data by searching each record and enter the data on the template.
5. Assess each record.
These steps should be modified to account for time constraints.
Organizational records are the source of organizational data. Records can be structured or unstructured. A structured record is formatted in such a way that makes it ready for analysis. This includes tables, spreadsheets, and models. Records such as memorandums, emails, videos, policies, and procedures are unstructured. Unstructured records require formatting before analysis can be conducted. In both cases, data sets are built from organizational records that can be integrated into the systematic review as independent data sets. As with scholarship, formatting records into a comprehensive data set can be a tedious process. The complexity and tedium involved in the formatting process increases the potential of introducing selection bias. Formatted data lends itself to easier access, analysis, and synthesis to create evidence for decision making. Because it is easier to access, analyze, and synthesize, decision makers will hypothetically build data sets primarily from formatted records. For this reason, it is incumbent on practitioners to consider bias in a critical appraisal of organizational data. Employing multiple appraisals for independent practitioners is one method for mitigating bias. In addition, applying the risk caveat provides a risk assessment of accepting bias.
Table 1. Record appraisal tool
|
Validity |
Reliability |
Rigor |
Transparency |
Total Score |
Source Total |
Record 1
|
|
|
|
|
|
|
Record 2
|
|
|
|
|
|
|
Record 3
|
|
|
|
|
|
|
Record 4
|
|
|
|
|
|
|
Source: courtesy of the author.
Table 1 provides an example of how to appraise each record and arrive at an overall score for organizational data. Each criterion is appraised on a scale chosen by the practitioner. A sum of the scores will result in a source score, which is to be incorporated into the overall Q score.
Appraising Human Sources
The concept used herein for appraising human sources is that since both the person and the data collected from that person introduce bias, both must be appraised. In addition to rigor, transparency, validity and reliability, the criterion for appraising a person includes measures of education and experience. Education and experience can be appraised with criteria used by human resources practitioners during a hiring process. For example, hierarchies are established that grant favorability for longevity in specific experiences and favorability for academic achievement. In this method of appraisal, the time continuum is the common thread (i.e., more time invested in either is better). Therefore, education and experience become exchangeable with time as a continuum. Human resource practitioners typically determine whether education or experience will be given priority based on tasks identified in a job description. Similarly, subject matter experts and stakeholders can be appraised based on the information they provide in answering research questions and the risk caveat. A sample of human qualification questions for assessing human sources is provided below. An appropriate rating scale is used to measure the levels of rigor, transparency, validity, and reliability associated with each question.
1. Does the person’s experience demonstrate rigor in performing technical and tactical task proficiency?
2. Does the person transparently divulge a comprehensive perspective?
3. Is the person’s knowledge such that they would be considered a valid stakeholder or subject matter expert?
4. Is the information provided reliable in terms of its accuracy?
The depth to which this path of inquiry is explored depends on the resources available.
In the following paragraphs, measures of education and experience as well as measures of risk are explained in detail. The logic behind appraising the person and the data collected from that person is to present a complete picture of where potential bias may originate. Hypothetically, assessing the data collected from a person without assessing that person’s education and experience would not reveal conflicts of interest or marginally qualified data inputs associated with that person. Contrariwise, assessing only the person would not reveal the bias introduced in the data set from creating usable formats from records.
Data from Human Sources
Data is collected from human sources by way of surveys, which include questionnaires, observations, and interviews. Each of these has advantages and disadvantages. One advantage of a questionnaire is efficiency, while a disadvantage is that responses are standardized, which creates a greater chance of skewing the final data set. As observations allow data to be collected in a natural environment, the approval required to conduct an observation can be difficult to obtain. The interview is the preferred interface for gathering data from a subject matter expert, as it affords open-ended questioning that can be modified during data collection. Information taken from surveys and observations is also measured with rating scales, which are best conceptualized during the data collection planning process.
Each individual’s human qualification questions are appraised separately, while questionnaires with sampled participants that ask the same questions can be batched and appraised as a group. Table 2 depicts an example of an appraisal of individuals and groups combined.
Table 2. Stakeholders
|
Validity |
Reliability |
Rigor |
Transparency |
Total Score |
Source Total |
Observations
|
|
|
|
|
|
|
Questionnaires
|
|
|
|
|
|
|
Librarian
|
|
|
|
|
|
|
Customer
|
|
|
|
|
|
|
Source: courtesy of the author.
Subject matter expertise is the professional experience and judgment of practitioners. The components of subject matter expertise that are to be evaluated include experience, decision-making history, and theories and philosophies. For example, asking how an expert gained their experience through employment, volunteering, or proximity observation provides initial context of the decision to be made. Based on these factors, the expected biases can be revealed. Similarly, education should be evaluated in terms of how it relates to the expected knowledge base gained and how it combines with experience.
Stakeholder input includes the values and concerns of people who may be affected by the decision. Hypothetically, there are two schools of thought on appraising stakeholders. The first operates under the opinion that stakeholder input should be brought into the decision-making process after conclusions have been drawn from the data—that is, after the evidence has shown compelling trends that lead to clear conclusions. In this view, the stakeholder has no influence in the analysis and synthesis of data and no input into the conclusions before they are drawn.
The second school of thought includes stakeholder input at key milestones throughout the process. This method tends to be difficult to manage when stakeholders are facing significant changes with clear winners and losers. In these instances, stakeholders may attempt to skew the data in order to create evidence contrary to a proposed change. Stakeholder input is a critical component of evidence in a systematic review, and as such, it is critical to recognize stakeholder biases that threaten the objectivity of any decision-making process. Hypothetically, stakeholder buy-in is facilitated by a recognition of their voice in the process, meaning that regardless of the process or the level of input, it is important that stakeholders recognize that their input is being considered.
Stakeholder input is assessed in two parts: the first involves an assessment of the person; and the second involves an assessment of the data collected from that person. This is shown in table 3, in which the subject matter expert and their associated interview are appraised independently. The scores for subject matter experts are then summed for an overall source score.
Table 3. Subject matter experts
|
Validity |
Reliability |
Rigor |
Transparency |
Total Score |
Source Total |
SME 1
|
|
|
|
|
|
|
Interview 1
|
|
|
|
|
|
|
SME 2
|
|
|
|
|
|
|
Interview 2
|
|
|
|
|
|
|
Source: courtesy of the author.
Conclusion
Whether the appraisal methodologies for a particular study are already well-established or developed specifically for that study, reducing bias can be accomplished during the appraisal process. The guidance outlined in this article provides practitioners a pathway to appraising evidence within the EBM framework and using the systematic review to integrate it with MJDM methodologies.
This article completes the present series on the integration of the EBM framework and the MJDM process. All of the models, instructions, and recommendations within the series are accessible through Marine Corps University Press and its online academic journal Expeditions with MCUP. The methodologies within this series began with research that demonstrated the feasibility of integrating the EBF into the MJDM process. This research presented the potential for extending the reach of Joint planning as an operational art through the use of systematic reviews. The systematic review brings scholarship, organizational data, stakeholder input, and subject matter expertise together in specific formats to supplement the operational designs, staff estimates, and operational assessments within the Joint planning process. The systematic review also introduces the methodologies for collecting and appraising data. These methods will be particularly useful in complex adaptive systems in which military and civil authority converge—specifically, in populace and resource control planning for civil affairs and in information campaign planning for psychological operations. Regardless of the type of operation or scope of the mission in which the operational arts of conflict are planned, the EBM-MJDM integration described in this series will enhance decision making at all levels.
Endnotes
- See David E. McCullin, “Exploring Evidence-Based Management in Military Planning Processes as a Critically Appraised Topic,” Expeditions with MCUP, 16 July 2021, https://doi.org/10.36304/ExpwMCUP.2021.04.
- See David E. McCullin, “The Integration of the Evidence-Based Framework and Military Judgment and Decision-Making,” Expeditions with MCUP, 10 November 2021, https://doi.org/10.36304/ExpwMCUP.2021.07.
- See David E. McCullin, “Integrating Information Literacy with the Evidence-based Framework and Military Judgment and Decision Making,” Expeditions with MCUP, 31 March 2023, https://doi.org/10.36304/ExpwMCUP.2023.04.
- Joseph R. Biden Jr., “Memorandum on Restoring Trust in Government through Scientific Integrity and Evidence-Based Policymaking,” White House, 27 January 2021.
- Eric Barends, Denise M. Rousseau, and Rob B. Briner, eds., CEBMa Guideline for Critically Appraised Topics in Management and Organizations (Amsterdam, Netherlands: Center for Evidence-Based Management, 2017), 3.
- Cynthia Grant and Azadeh Osanloo, “Understanding, Selecting, and Integrating a Theoretical Framework in Dissertation Research: Creating the Blueprint for Your ‘House’,” Administrative Issues Journal: Connecting Education, Practice, and Research 4, no. 2 (2014): 13, https://doi.org/10.5929/2014.4.2.9.
- Eric Barends, Denise M. Rousseau, and Rob B. Briner, Evidence-Based Management: The Basic Principles (Amsterdam, Netherlands: Center for Evidence-Based Management, 2014), 201.
- Barends, Rousseau, and Briner, Evidence-Based Management, 5.
- Harold E. Briggs and Bowen McBeath. “Evidence-Based Management: Origins, Challenges, and Implications for Social Service Administration,” Administration in Social Work 33, no. 3 (2009): 245–48, https://doi.org/10.1080/03643100902987556.
- Grant and Osanloo, “Understanding, Selecting, and Integrating a Theoretical Framework in Dissertation Research,” 13.
- Herbert A. Simon, The Sciences of the Artificial (Cambridge, MA: MIT Press, 1968), 178.
- Grant and Osanloo, “Understanding, Selecting, and Integrating a Theoretical Framework in Dissertation Research,” 16–17.
- David E. McCullin, “The Impact of Organizational Complex Adaptive System Constraints on Strategy Selection: A Systematic Review of the Literature” (DM diss., University of Maryland Global Campus, 2020), 1–2.
- See McCullin, “The Integration of the Evidence-Based Framework and Military Judgment and Decision-Making.”
- Joint Planning, Joint Publication 5-0 (Washington, DC: Joint Chiefs of Staff, 2017).
- Barends, Rousseau, and Briner, Evidence-Based Management, 207.
- Barends, Rousseau, and Briner, Evidence-Based Management, 7.
- See McCullin, “Exploring Evidence-Based Management in Military Planning Processes as a Critically Appraised Topic”; and McCullin, “The Integration of the Evidence-Based Framework and Military Judgment and Decision-Making.”
- Barends, Rousseau, and Briner, Evidence-Based Management, 1.
- Barends, Rousseau, and Briner, Evidence-Based Management, 12–14.
- Amos Tversky and Daniel Kahneman, “Availability: A Heuristic for Judging Frequency and Probability,” Cognitive Psychology 5, no. 2 (September 1973): 207–32, https://doi.org/10.1016/0010-0285(73)90033-9.
- Phanish Puranam et al., “Modeling Bounded Rationality in Organizations: Progress and Prospects,” Academy of Management Annals, 9, no. 1 (2015), 337–92, https://doi.org/10.5465/19416520.2015.1024498.
- Tversky and Kahneman, “Availability.”
- “Decision-Making,” Psychology Today, accessed 29 August 2023.
- Tversky and Kahneman, “Availability.”
- McCullin, “The Impact of Organizational Complex Adaptive System Constraints on Strategy Selection,” 1–2.
- Guy ParĂ© et al., “Synthesizing Information Systems Knowledge: A Typology of Literature Reviews,” Information & Management 52, no. 2 (March 2015): 183–99, https://doi.org/10.1016/j.im.2014.08.008.
- David Gough, “Weight of Evidence: A Framework for the Appraisal of the Quality and Relevance of Evidence,” Research Papers in Education 22, no. 2 (2007): 218–28, https://doi.org/10.1080/02671520701296189.
- See McCullin, “Integrating Information Literacy with the Evidence-based Framework and Military Judgment and Decision Making.”